Rudder Admin
Voice-Controlled Amenities for Enhanced Hotel Guest Experience
Voice-Controlled Amenities for Enhanced Hotel Guest Experience
In the hospitality sector, delivering exceptional guest experiences is a top priority. One hotel chain recognized an opportunity to enhance its offerings through voice-enabled technology. They partnered with us to implement a wake word detection system and voice-activated concierge services. The goal was to elevate convenience and satisfaction by enabling guests to control room amenities like lighting, temperature, and entertainment via voice commands. This technical blog post will dive into the details of the wake word detection system developed by Rudder Analytics, exploring the approaches used to ensure accurate speech recognition across diverse acoustic environments, user voices, and speech patterns.
Wake Word Detection
Wake word detection, also known as keyword spotting, is a critical component of voice-enabled systems that allow users to activate and interact with devices or applications using predefined voice commands. This technology is crucial in various applications, including virtual assistants, smart home devices, and voice-controlled interfaces.
The primary objective of wake word detection is to continuously monitor audio streams for the presence of a specific wake word or phrase. After detecting the wake word, the system activates and listens for subsequent voice commands or queries. Effective wake word detection systems must balance accuracy, computational efficiency, and power consumption.
A brief overview of the process:
The process begins when the user speaks to the device/application. The words are captured as an audio input.
Next comes the feature extraction where specific characteristics are extracted from the sound of the user’s voice that will help recognize the wake word.
Now, the device/application turns these features into embedding representation, like a unique digital fingerprint that represents the wake word’s sound pattern.
This is where the pre-trained model comes into play. Before the device/application listens to the user, it is trained on many examples to learn what the wake word sounds like.
This model is fine-tuned with target keyword examples (the actual wake words it needs to listen for) and non-target examples (all the other words that aren’t the wake word).
The fine-tuned model then captures only the wake word while neglecting the other non-target words.
Challenges to Tackle
The main challenge was to develop a wake word detection system that accurately recognizes specific commands within a continuous audio stream. This task was complicated by the need for the system to perform reliably across various acoustic settings, from quiet rooms to those with background noise or echoes. Additionally, the system had to be versatile enough to recognize spoken commands by a wide array of users, each with their unique voice, accent, and speech pattern.
Crafting Our Solution
Few-Shot Transfer Learning Approach
Few-shot transfer learning is a technique that can enable machine learning models to quickly adapt to new tasks using only a limited number of examples. The approach builds upon extensive prior training on related but broad tasks, allowing models to leverage learned features and apply them to new, specific challenges with minimal additional input.
This strategy is particularly valuable in scenarios where data is scarce. By enhancing model adaptability and efficiency, this technique offers a realistic solution to data scarcity in natural language processing. The ability of few-shot transfer learning to empower machine learning models to generalize from limited data has significant practical applications, making it an increasingly popular research topic in the field of artificial intelligence.
Model Fine Tuning
1. Starting With a Foundation
Our system begins with a pre-trained multilingual embedding model. This is a base model that’s already been trained on a vast array of languages and sounds, giving it a broad understanding of speech patterns.
Pre-trained Multilingual Embedding Model
Our approach leveraged a Deep Neural Network (DNN)-based, pre-trained multilingual embedding model. This model was initially trained on 760 frequent words from nine languages, drawing from extensive datasets such as:
MLCommons Multilingual Spoken Words: Contains more than 340,000 keywords, totaling 23.4 million 1-second spoken examples (over 6,000 hours)
Common Voice Corpus: 9,283 recorded hours with demographic metadata like age, sex, and accent. The dataset consists of 7,335 validated hours in 60 languages.
Google Speech Commands for background noise samples: 8.17 GiB audio dataset of spoken words designed to help train and evaluate keyword spotting systems.
This rich training background laid a foundation for the system’s language and accent inclusivity.
2. Selective Hearing
The fine-tuning process involves teaching the model to focus on a small set of important sounds – the wake words. Fine-tuning the model for a new wake word using few-shot transfer learning is achieved by updating the model’s weights using a small dataset of audio recordings containing the new wake word.
With the hotel’s custom needs in mind, we fine-tuned the model with just five custom target keyword samples as training data. This method allowed us to fine-tune a five-shot keyword spotting context model, enabling the pre-trained model to generalize over new data categories swiftly.
3. Distinguishing the Target
It’s not just about knowing the wake word but also about knowing what it’s not. An unknown keywords dataset of 5,000 non-target keyword examples was used to maintain the ability of the few-shot model to distinguish between the target keyword and non-target keywords.
4. Tailored Adjustments
The pre-trained model was adjusted incrementally, learning to recognize the wake word more accurately from the examples provided. This involved tweaking the internal settings, or parameters, of the model to minimize errors. The fine-tuning process typically employs a variant of stochastic gradient descent (SGD) or other optimization algorithms.
5. Testing and Retesting
After each adjustment, the model was tested to see how well it can distinguish the wake word from other sounds. It’s a cycle of testing, learning, and improving.
6. Optimizing for Real World Use
During fine-tuning, the model was introduced to variations of the wake word as it might be spoken in different accents, pitches, or speech speeds, ensuring the model can recognize the wake word in diverse conditions. This was done by using techniques like data augmentation and noise addition.
7. Reducing False Triggers
A crucial part of fine-tuning is to reduce false positives—times when the device wakes up but shouldn’t. This involves adjusting the model so that it becomes more discerning and able to tell apart similar words or sounds from the actual wake word.
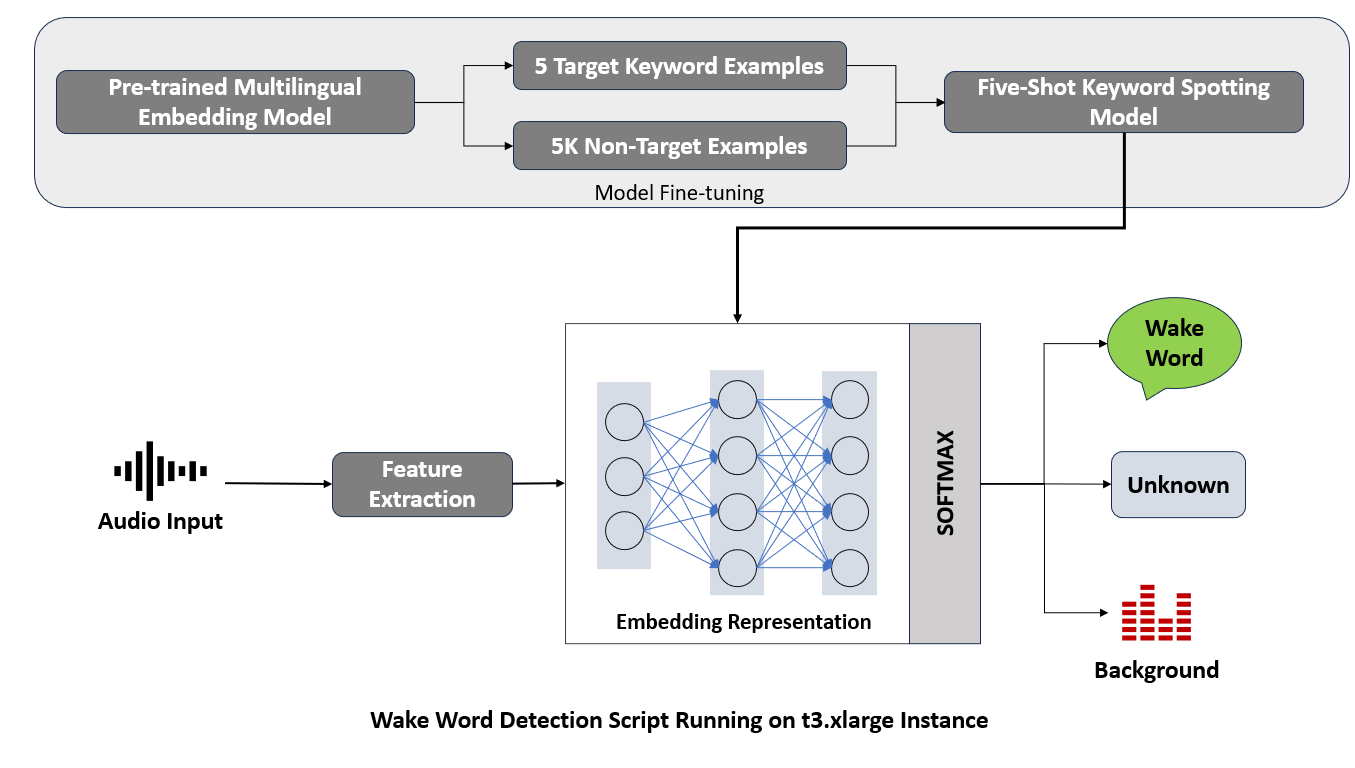
Fine-tuned Wake Word Detection Model
1. Audio Input and Feature Extraction
At the start of the wake word detection pipeline, audio input is received and passed through a feature extraction process. This step is crucial for transforming raw audio waveforms into a structured format that the neural network can interpret. Feature extraction algorithms focus on isolating the most relevant aspects of the audio signal, such as frequency and amplitude, which are informative of the content within the audio.
2. Neural Network and Embedding Representation
The extracted features are then input into a neural network, which acts as the engine of the wake word detection system. The network maps the features to an embedding space, where the learned representations are optimized to cluster target wake words close together while distancing them from non-target sounds and words.
3. The Softmax layer
The use of a softmax layer is standard in classification tasks. However, in the context of wake word detection, the softmax layer presents a unique challenge. It needs to classify inputs into one of three categories: the wake word, unknown words, or background noise. The softmax layer must be finely tuned to ensure that it can confidently distinguish between these categories, which is critical for reducing both false positives and negatives.
4. Real-time Processing
An efficient sliding window mechanism was implemented to enable the real-time analysis of continuous audio streams, ensuring prompt system responsiveness with minimal latency.
5. Deployment on a Cloud Instance
Once the model is trained and validated, it’s deployed to a cloud-based service running on a t3.xlarge instance. This selection of cloud computing resources ensures that the wake word detection script has access to high performance and scalability to handle real-time audio processing without significant latency.
Measurable Impact and Beyond
The implementation of this system had a clear impact, achieving an accuracy of 97% in wake word detection and a remarkable 99.9% uptime during stress testing and performance evaluations. This reliability ensured the system’s scalability and dependability, critical factors in a hotel environment where downtime can significantly affect guest satisfaction.
The most telling outcome was the 23% increase in guest satisfaction scores following the system’s implementation. This surge in guest approval underscored the system’s effectiveness in enhancing the overall stay experience, affirming the value of integrating AI and ML technologies in service-oriented industries.
Our wake word detection system ensured high accuracy, decreased the occurrence of false positives, and operated with reduced latency, facilitating immediate and correct command detection, and thereby considerably improving the overall user experience.
Conclusion
In wrapping up this project on voice-controlled hotel room amenities, it’s clear that our technical efforts and the application of AI and ML have significantly improved how customer service can be delivered. This work highlights the practical benefits of leveraging advanced technologies to make everyday interactions more user-friendly and efficient. At Rudder Analytics, our focus remains on exploring the potential of AI and ML to contribute to progress and achieve high standards in various fields.
Elevate your projects with our expertise in cutting-edge technology and innovation. Whether it’s advancing voice-controlled system capabilities or pioneering new tech frontiers, our team is ready to collaborate and drive success. Join us in shaping the future—explore our services, and let’s create something remarkable together. Connect with us today and take the first step towards transforming your ideas into reality.
Drop by and say hello! Website LinkedIn Facebook Instagram X GitHub
Streamlining Medical Transcription with Speaker Diarization
Streamlining Medical Transcription with Speaker Diarization
In the modern era of digital communication, the need for accurate and efficient transcription of conversations has become greatly important across various industries. However, manually transcribing lengthy conversations, particularly those involving multiple speakers, can be daunting, often plagued by errors, inefficiencies, and time-consuming processes. Enter speaker diarization technology, an innovative solution that promises to transform how we approach conversation transcription.
Understanding Speaker Diarization
Speaker diarization is an advanced technology that aims to identify and label different speakers in an audio recording automatically. Unlike traditional transcription methods, speaker diarization goes beyond mere text conversion by providing speaker-specific information, such as the start and end times for each speaker’s utterances. This technology is precious in scenarios involving multiple speakers, such as meetings, interviews, legal proceedings, or medical procedures.
Speaker diarization relies on sophisticated algorithms and machine learning techniques to analyze the audio stream, detect speaker changes, and associate each speech segment with a unique speaker label. By doing so, it enables accurate and comprehensive transcription, ensuring that every word spoken is accurately attributed to the correct speaker.
Optimizing Operating Room Transcription
In the healthcare domain, accurate and comprehensive medical documentation is of utmost importance, as it directly impacts the quality of patient care and safety. Our client, a leading provider of surgical services, recognized the immense potential of speaker diarization technology in optimizing operating room transcription processes.
Before implementing our solution, the client faced significant challenges in accurately transcribing surgical procedures, where multiple healthcare professionals communicate simultaneously during the operation. Manual transcription was not only time-consuming but also prone to errors, hindering post-operative analysis and potentially compromising the integrity of medical records.
Understanding the Challenge
Developing an effective speaker diarization system for conversation transcription presented several complex challenges, including:
Speaker Identification: The system needed to accurately identify and differentiate between multiple speakers, such as surgeons, nurses, and anesthesiologists, even in the acoustically challenging environment of an operating room.
Dynamic Acoustic Conditions: Surgical rooms are inherently noisy and filled with unexpected sounds, from medical equipment to overlapping conversations. The diarization system had to be resilient and adaptable to these conditions, ensuring consistent accuracy.
Temporal Precision: It was crucial for the system to not only recognize different speakers but also to precisely log the start and end times of each speaker’s contributions, providing a detailed and chronological account of the spoken dialogue.
Crafting the Solution
Developing an effective speaker diarization system that meets the intricate demands of surgical procedure transcriptions involves a comprehensive, multi-stage process. At the heart of our approach lies utilizing the Kaldi Automatic Speech Recognition (ASR) toolkit with its callhome recipe. We have used a Time-Delay Neural Network (TDNN) based x-vector model in our approach. Kaldi is celebrated for its versatility and strength in tackling a wide array of speech recognition tasks, making it an ideal choice for our ambitious project.
If you are interested in the codebase, check out our GitHub repository
Feed-Forward Deep Neural Network
At the core of our speaker diarization system lies a robust neural network architecture designed to extract speaker embeddings from variable-length acoustic segments. This feed-forward Deep Neural Network (DNN), illustrated in Figure 1 (source), employs a carefully crafted combination of layers to capture the intricate characteristics of speech signals.
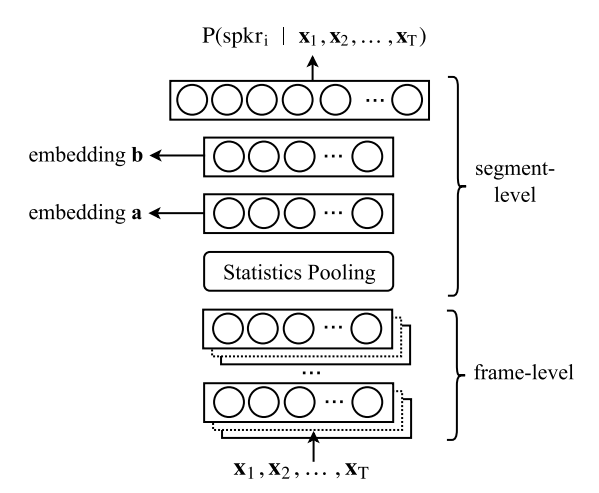
Feature Extraction
The neural network’s input consists of Mel-Frequency Cepstral Coefficient (MFCC) features, a widely adopted representation of the speech signal’s spectral characteristics. These features are extracted using a 20ms frame length and normalized over a 3-second window, ensuring consistent and robust feature representations.
Frame-Level Processing
The initial five layers of the network operate at the frame level, leveraging a time-delay architecture to model short-term temporal dependencies effectively. Unlike traditional stacked frame inputs, this architecture seamlessly incorporates temporal context by design, enhancing the network’s ability to capture the intricate dynamics of speech signals.
Statistics Pooling
The network’s statistics pooling layer is pivotal in condensing the frame-level representations into segment-level statistics. By aggregating the output of the final frame-level layer, this layer computes the mean and standard deviation of the input segment, encapsulating its essential characteristics.
Segment-Level Processing
The segment-level statistics, comprising the mean and standard deviation, are concatenated and passed through two additional hidden layers. These layers further refine and enrich the extracted representations, preparing them for the final speaker embedding generation.
Output Layer
The softmax output layer serves as the culmination of the network’s processing pipeline. It receives the refined segment-level representations and generates the final speaker embeddings, which encapsulate the distinguishing characteristics of each speaker.
Architectural Dimensions
Excluding the softmax output layer, the neural network boasts a substantial 4.4 million parameters, enabling it to capture intricate patterns and nuances within the speech data.
Diarization Process in Kaldi
After designing the model architecture and training the model using callhome recipe, we started the speaker diarization process in Kaldi.
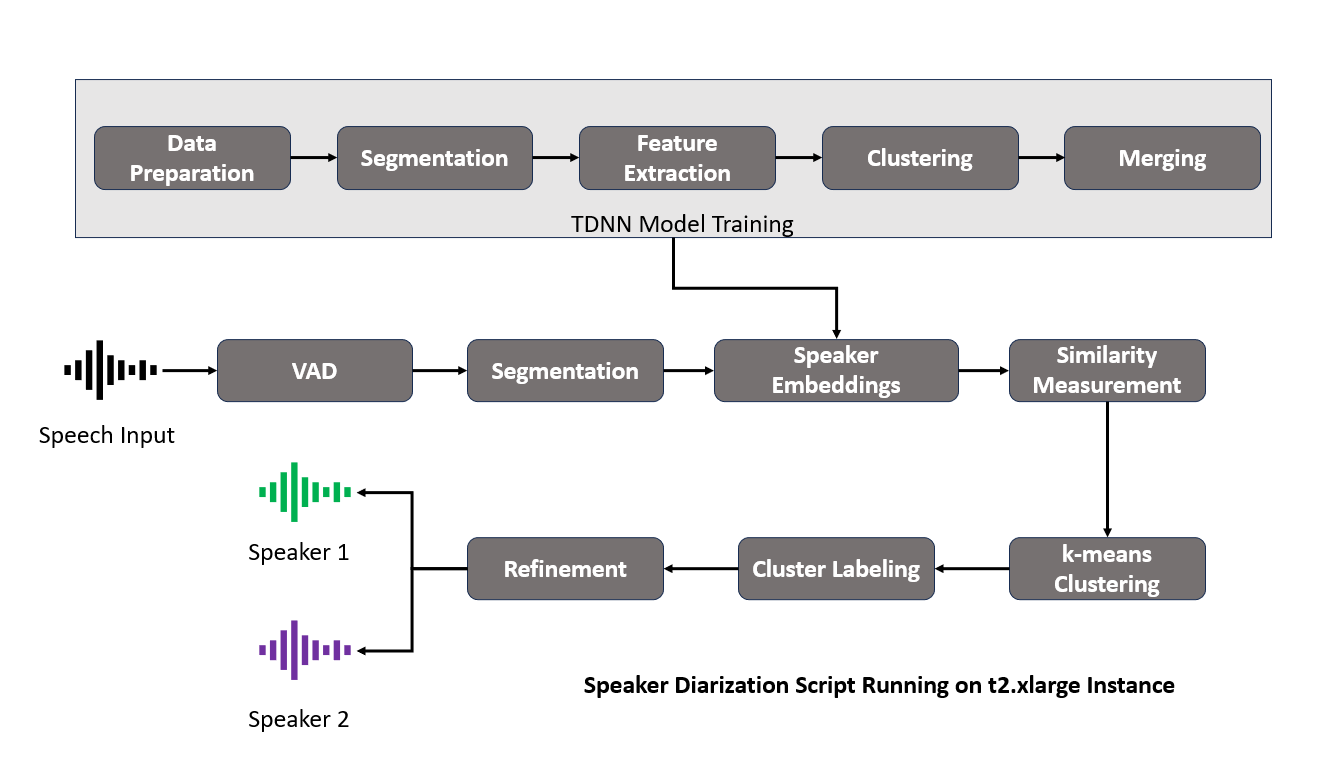
Step 1: Data Preparation
We began by organizing our audio data for Kaldi’s processing. This involves creating two essential files: the wav.scp, which maps each audio file to its location, and the spk2utt file which maps the speaker to the utterance.
Step 2: Feature Extraction
For feature extraction, we used Kaldi’s make_mfcc.sh and prepare_feats.sh scripts. Employing Mel Frequency Cepstral Coefficients (MFCCs) and Cepstral Mean and Variance Normalization (CMVN), we captured the unique acoustic signatures of each speaker, laying a robust foundation for the intricate process of speaker differentiation.
Step 3: Creating the Segments File
After feature extraction, the next step was to generate the segments file, detailing the start and end times of speech within the input file. The script: compute_vad_decision.sh was used to identify speech segments, crucial for accurate diarization.
Step 4: X-Vector Creation
With our features ready, we created x-vectors using the extract_xvectors.sh script from Kaldi. These vectors serve as compact representations of each speaker’s characteristics within the audio, essential for differentiating between speakers.
Step 5: PLDA Scoring
Next, we applied Probabilistic Linear Discriminant Analysis (PLDA) to score the similarity between pairs of x-vectors. PLDA scores were calculated using Kaldi’s score_plda.sh script. This statistical method helps in modeling the speaker-specific variability and is instrumental in the clustering phase, where speaker embeddings are categorized.
Step 6: Clustering
We then used the PLDA scores as a basis for clustering the x-vectors. cluster.sh script from Kaldi groups the vectors based on their similarity, effectively organizing the audio segments by speaker identity. The goal is to ensure that segments from the same speaker are grouped accurately.
Step 7: Refinement
After initial clustering, we employed PLDA again using cluster.sh script in a re-segmentation step to refine the diarization output. By assessing PLDA scores for shorter segments or frames, we could make detailed adjustments to speaker segment boundaries, enhancing the precision of the diarization results.
Finally, we combined the diarization results with the Automatic Speech Recognition system to generate the output.
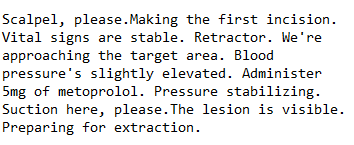
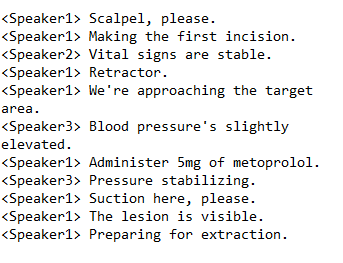
Realizing the Impact
The implementation of this sophisticated speaker diarization system led to a notable improvement in the transcription process. With a Diarization Error Rate (DER) reduced to 4.3%, the system demonstrated remarkable accuracy in distinguishing between speakers. This advancement yielded significant operational efficiencies, notably a 40% reduction in the time required for post-operative review and analysis. Moreover, the integration of this technology into Electronic Health Record (EHR) systems resulted in a 30% decrease in data entry errors, ensuring more accurate and synchronized patient records.
Conclusion: The Intersection of AI and Healthcare
Rudder Analytics’ integration of speaker diarization in healthcare highlights our commitment to bridging sophisticated technology with practical needs. Our primary objective is to accurately capture and document every word spoken in the operating room, aiding in precise surgical transcriptions. This initiative is part of our broader mission to drive advancements in patient care. By harnessing the power of AI and data analytics, we aim to solve intricate challenges, underscoring our dedication to thoughtful innovation within the healthcare domain. Through this endeavor, we seek to showcase the pivotal role that advanced technologies can play in enhancing the quality of care provided to patients.
As technology continues to evolve, the applications of speaker diarization extend far beyond the healthcare industry. Businesses, legal firms, media organizations, and various other sectors can benefit from this technology, enabling accurate and efficient transcription of multi-speaker conversations, ultimately driving productivity, enhancing decision-making processes, and fostering better collaboration.
Elevate your projects with our expertise in cutting-edge technology and innovation. Whether it’s advancing transcription capabilities or pioneering in new tech frontiers, our team is ready to collaborate and drive success. Join us in shaping the future—explore our services, and let’s create something remarkable together. Connect with us today and take the first step towards transforming your ideas into reality.
Drop by and say hello! Medium LinkedIn Facebook Instagram X GitHub
Enhancing Digital Communication with Text-to-Speech Technologies
Enhancing Digital Communication with Text-to-Speech Technologies
In the dynamic world of digital communication, messaging apps play a crucial role in connecting people globally. The introduction of sophisticated Text-to-Speech (TTS) systems for these messaging apps marks a significant advancement. This article explores how Rudder Analytics collaborated with a leading messaging application developer to improve user accessibility and engagement. The aim was to make digital communication more inclusive and provide a more immersive experience for a varied audience.
The Challenge: Crafting Natural-Sounding Speech
Traditional TTS systems, while impressive, often fall short of capturing the full range and expressiveness of human speech. Our challenge was to develop a system that could convert text to speech smoothly, focusing on achieving a natural sound. Our goal was to closely mimic human speech’s pitch, rhythm, and emphasis variations, retaining the emotional and contextual richness that characterizes personal communication.
A crucial part of this challenge was ensuring the system could accurately pronounce a wide range of words, including common language, specific proper names, acronyms, and jargon, all within the correct context. This precision was essential for a smooth and accurate communication experience. We aimed to narrow the gap between synthesized and natural speech, making every generated speech sound as authentic as a real conversation.
Selecting the Right Tools: Coqui TTS and Glow-TTS
To effectively tackle the complexities of this challenge, a strategic approach was necessary. This involved the careful selection of tools and architectural frameworks that could fully leverage the latest technological advancements.
We chose Coqui TTS for its extensive language support and adaptability in fine-tuning and training across various linguistic contexts. Alongside, we utilized the Glow-TTS architecture, known for its efficient flow-based generative model for speech synthesis. This combination was key in producing expressive and lifelike speech, capturing the nuanced cadences and inflections of human conversation.
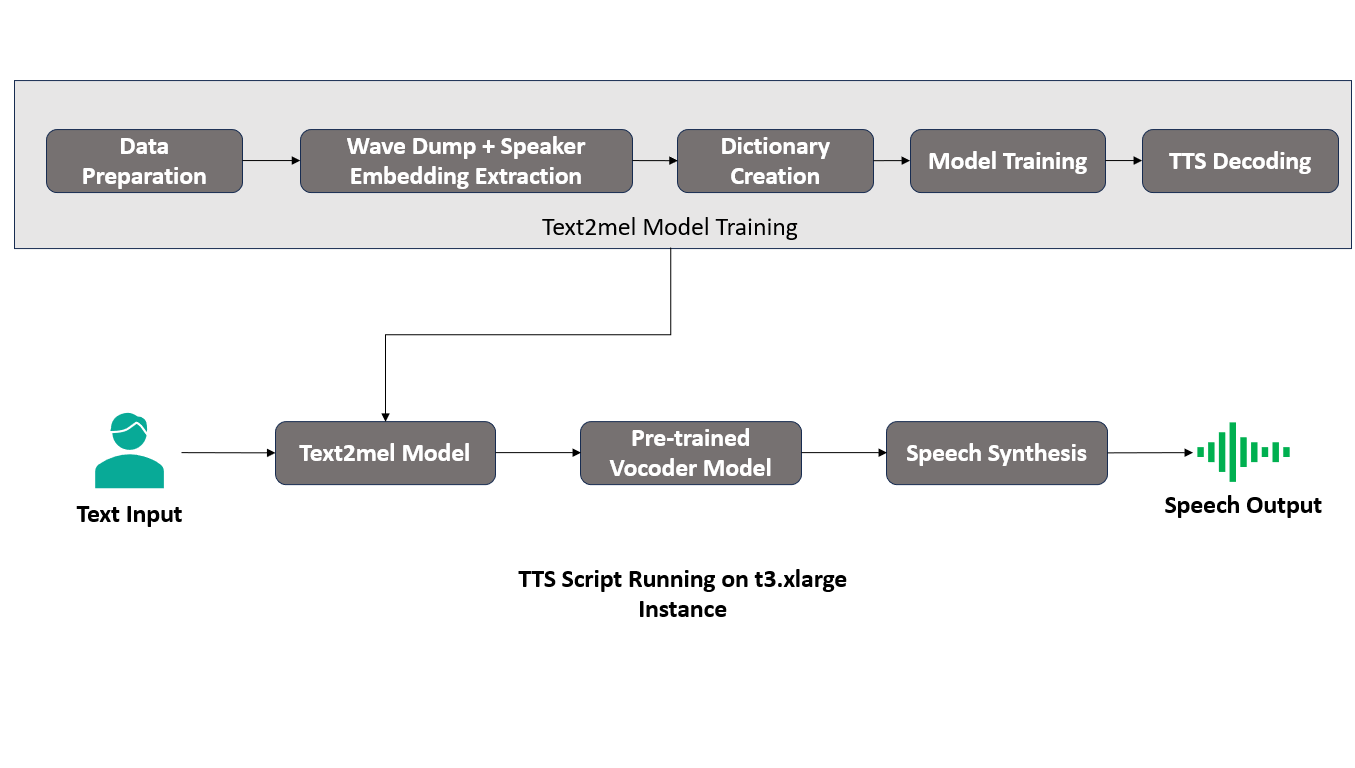
Building a Foundation: A Diverse and Rich Dataset
The success of AI technologies heavily relies on the quality and diversity of the training data. For our TTS system, we compiled a comprehensive dataset that included a wide range of text inputs and corresponding speech outputs, ensuring linguistic variety. This variety was crucial for training our model to handle the complexities of spoken language, making the synthesized speech sound natural and contextually appropriate.
Data Preparation and Optimization
We meticulously prepared our data, focusing on restructuring, formatting, and normalization to optimize it for model training. This preparation was vital for addressing the natural variability in language and enhancing the model’s learning efficiency. Phonetic transcription played a key role in improving the model’s pronunciation accuracy, bringing us closer to achieving our goal of natural-sounding speech.
Advanced Model Training and Real-Time Monitoring
Leveraging the computational capabilities of the NVIDIA A10 GPU, we undertook intensive training of our TTS model. Real-time monitoring through Tensorboard provided critical insights into the model’s performance, enabling continuous refinement and optimization. This combination of cutting-edge hardware and sophisticated monitoring tools allowed us to continuously refine and enhance our model.
Measuring Success: Outcomes and Feedback
The TTS system’s effectiveness was confirmed by a low Word Error Rate (WER) of 3%, indicating a high level of accuracy in speech reproduction. However, the most significant measure of success was the positive feedback from users, with 68% finding the synthesized speech to be more natural and engaging. The system’s multilingual capabilities further extended its reach and applicability.
A particularly noteworthy impact of this project was the increased engagement from users with visual impairments, highlighting our contribution to digital inclusivity. The engagement from visually impaired users rose from 5% to 15% of the total platform engagement, underscoring the importance of accessible digital platforms.
Conclusion: A Commitment to Accessible Communication
This project exemplifies the potential of TTS technologies to transform digital communication, making it more inclusive and engaging. At Rudder Analytics, we are committed to using advanced technologies to improve digital inclusivity and engagement. Through our efforts, we strive to create digital environments that are accessible and welcoming to all users, reflecting our commitment to inclusivity and user-centric solutions.
Elevate your projects with our expertise in cutting-edge technology and innovation. Whether it’s advancing speech synthesis capabilities or pioneering in new tech frontiers, our team is ready to collaborate and drive success. Join us in shaping the future—explore our services, and let’s create something remarkable together. Connect with us today and take the first step towards transforming your ideas into reality.
Innovating Legal Transcriptions with Custom German ASR Solutions
Innovating Legal Transcriptions with Custom German ASR Solutions
In the rapidly advancing digital era, the legal profession confronts unique challenges, particularly in ensuring transcription accuracy and clarity. At Rudder Analytics, we identified a pressing need within a distinguished German law firm, which was battling the constraints of conventional transcription methods. These challenges extended beyond mere efficiency, impacting the fundamental aspects of integrity and confidentiality in legal communications. Consequently, acknowledging the limitations of existing solutions, we embarked on a mission to develop an innovative, secure, and precise transcription system, specifically tailored to the nuanced demands of the legal domain.
Beyond Standard ASR Solutions
Our process commenced with a deep understanding of our client’s distinct requirements. Despite the sophistication of available Automatic Speech Recognition (ASR) technologies, they were unsuitable. They could not provide the essential data control and privacy required in legal contexts. This realization led us to the necessity of creating a custom-built ASR system, one that could be intricately tailored to meet the firm’s specific needs, thereby ensuring the absolute confidentiality of all transcription endeavors.
Engineering a Customized Solution
Our search for the ultimate ASR solution guided us to the Kaldi toolkit, celebrated within the open-source community for its robustness and versatility. Kaldi’s exceptional adaptability made it the ideal foundation. It made it possible to construct a system capable of handling the complexities of German legal discourse. Kaldi’s extensive capabilities include a wide range of modular tools for speech-processing tasks. We leveraged them to design an ASR system that would meet and exceed our client’s stringent expectations.
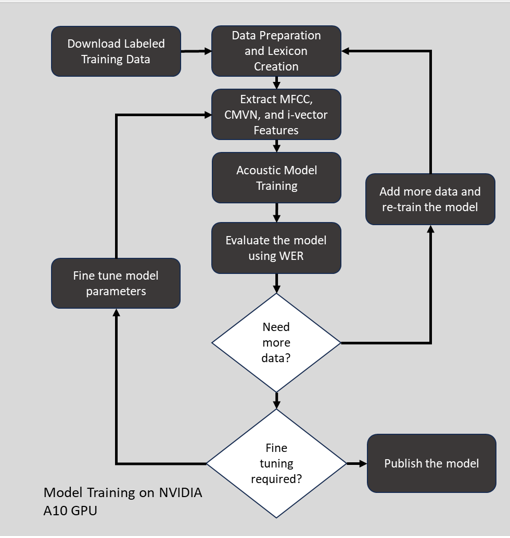
Quality Training Data: The Key to Precise Legal Transcriptions
The effectiveness of any ASR system is intrinsically linked to the caliber of its training data. We delved into the vast archives of the Tuda de and Mozilla Commonvoice datasets, extracting an extensive array of German audio samples. This diverse compilation of recordings covers various dialects and speech contexts. It provides the indispensable raw material needed to develop an acoustic model. A model, which is capable of accurately interpreting the specialized language of the legal sector.
Enhancing Data Quality: The Preprocessing Phase
Before initiating the training phase, our audio data underwent a comprehensive preprocessing phase. This vital step involved employing advanced techniques to enhance data quality, including sophisticated noise reduction, clarity improvement, and volume normalization. We utilized state-of-the-art algorithms for Mel-frequency Cepstral Coefficients (MFCC). These represent the short-term power spectrum of sound. Additionally, we employed Cepstral Mean and Variance Normalization (CMVN) to normalize speech features. Lastly, we used i-vector extraction to capture speaker and session variability. This ensured our model was trained on the highest quality audio features available.
Mastering the Acoustic Model: Capturing Speech Nuances
The heart of our ASR system was the acoustic model, meticulously crafted and trained using NVIDIA’s A10 GPU. We chose the Time Delay Neural Network (TDNN) architecture for its unparalleled ability to process the temporal variations in speech. TDNN, a type of deep neural network, excels in recognizing patterns over varying time scales, making it particularly effective for speech tasks. Through intensive training on our carefully curated dataset, we fine-tuned the model. It discerns the subtle intricacies of the German language used in legal settings. This ensures unmatched transcription accuracy.
Evaluating the Acoustic Model
An essential step in our implementation process involved thorough system testing and evaluation. We evaluated the model’s performance using Word Error Rate (WER), a widely used metric in speech recognition. WER helped us gauge how frequently the model inaccurately predicted phonemes. WER is crucial for assessing Automatic Speech Recognition (ASR) systems, providing a measurable indicator of transcription accuracy. It is calculated by comparing the ASR system’s output to a reference transcription, considering the number of substitutions, deletions, and insertions required to align the system’s output with the reference.
Achieving Transcription Accuracy: Integrating Language Models and Decoding Graphs
We incorporated a sophisticated language model along with a comprehensive decoding graph. The language model, crucial for understanding the grammatical and syntactic structures of the German language, refined the system’s word sequence predictions. Simultaneously, our decoding graph, a complex structure that evaluates potential word sequences against the audio input, analyzed these sequences, ensuring the final transcriptions were not only precise but also coherent.
During the decoding process, the sophisticated acoustic model would first map raw audio to a lattice of phoneme predictions based on its training. The language model could then refine and contextualize these into valid word sequence hypotheses, leveraging encoded rules about German linguistic patterns. Finally, the decoding graph would prune and rank these word hypotheses against the lexicon constraints to output the most statistically likely transcription.
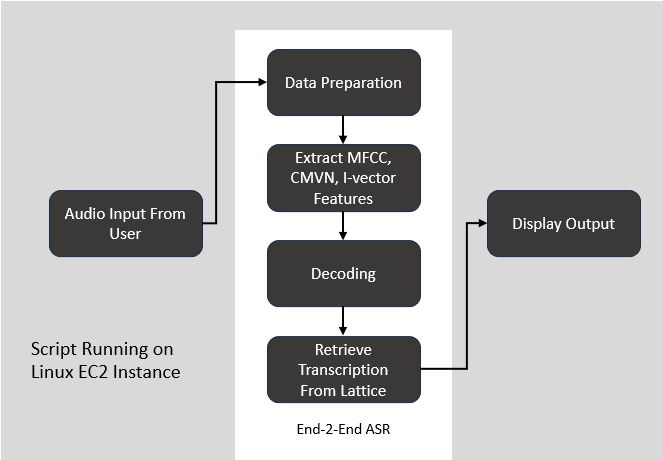
Enhancing Legal Transcription Standards
The introduction of our custom German ASR system marked a significant milestone for our client. Achieving an impressive Word Error Rate (WER) of 3.2% under standard conditions and demonstrating resilience with a 5.2% WER in challenging acoustic situations. Our system established new benchmarks for transcription accuracy in the legal arena. Its proficiency in processing specialized legal vocabulary renders it an indispensable tool for our client.
Moreover, our system facilitated significant cost savings, reducing manual transcription expenses by up to 70% and substantially boosting staff morale. We remained steadfast in our commitment to data protection and privacy, ensuring our system adhered to the most stringent privacy regulations, achieving 100% compliance. The system’s consistent performance across various accents, speaking styles, and audio quality levels cemented its critical role in our client’s operational framework.
Charting the Future with Cutting-edge Legal Technology
Our German ASR system’s success proves AI and machine learning can transform industries. This is especially true for the nuanced and sensitive legal industry. This project met our client’s immediate transcription needs while leading the pathway for integrating advanced technological solutions into traditional practices. As we strive to advance the frontiers of AI and machine learning, our initiative stands as a guiding light. It demonstrates how cutting-edge technology can greatly improve professional efficiency, security, and adaptability. This heralds a new era in seamlessly integrating technology and law.
Elevate your projects with our expertise in cutting-edge technology and innovation. Whether it’s advancing legal documentation or pioneering in new tech frontiers, our team is ready to collaborate and drive success. Join us in shaping the future—explore our services, and let’s create something remarkable together. Connect with us today and take the first step towards transforming your ideas into reality.
A Deep Dive into Phoneme-Level Pronunciation Assessment
A Deep Dive into Phoneme-Level Pronunciation Assessment
In the rapidly evolving digital education domain, our team at Rudder Analytics embarked on a pioneering project. We aimed to enhance language learning through cutting-edge AI and machine learning technologies. Partnering with a premier language learning platform, we sought to address a significant challenge in the field: providing detailed and actionable feedback on pronunciation at the phoneme level, a critical aspect of mastering any language. This case study delves into the sophisticated technical landscape we navigated to develop an advanced phoneme-level pronunciation assessment tool, showcasing our data analytics, engineering, and machine learning expertise.
Navigating the Challenge: Beyond Conventional Solutions
The initial challenge was the limitations of out-of-the-box pronunciation scoring APIs provided by major cloud services like GCP, Azure, and AWS. These services, while robust, fell short of delivering the granular level of detail required for effective pronunciation assessment. To overcome this problem, the decision was made to construct a bespoke model that could meet the specific needs of the platform.
Our objective was clear: to architect a solution that transcends these limitations, enabling a more personalized and impactful learning experience.
Holistic Approach: Integrating Advanced Algorithms with Linguistic Insights
Our strategy was anchored in a holistic approach, merging advanced machine learning techniques with deep linguistic insights to achieve higher accuracy in pronunciation assessment.
If you are interested in the codebase, check out our GitHub repository
Goodness of Pronunciation (GOP)
A cornerstone of our approach was the implementation of the Goodness of Pronunciation (GOP) metric. GOP, a posterior probability variant, is a quantitative measure of pronunciation accuracy at the phoneme level. It’s an important tool for identifying mispronunciations, enabling targeted feedback for language learners. GOP is used to evaluate the system’s performance in recognizing and scoring the pronunciation of a given utterance.
The Strategic Employment of Kaldi ASR Toolkit
Kaldi, an open-source ASR framework, stands at the core of our solution. Renowned for its flexibility and efficiency in handling speech recognition tasks, Kaldi offers a range of recipes for tailoring acoustic models to specific needs. Our choice to utilize Kaldi was driven by its comprehensive feature set and its ability to be customized for phoneme level detection, a critical requirement for our project.
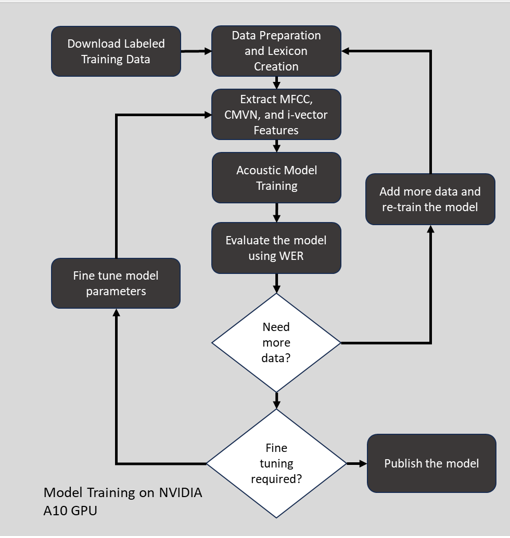
Data Collection and Preparation
The foundation of our solution was a robust data infrastructure, engineered to handle vast datasets. We utilized the Librispeech dataset, a comprehensive collection of English language audio files. It contains over 1000 hours of speech, recorded by 2,484 speakers, and has been designed to be representative of the different accents and dialects of spoken English. These recordings were made using high-quality microphones and a sound-treated recording environment to ensure high-quality audio.
This dataset contains labeled audio data. We also collected the pronunciation lexicon which included words and their corresponding sequences of phonemes, essential for training our model to recognize and evaluate the smallest sound units in speech.
Major Components
In Kaldi, when computing Goodness of Pronunciation (GOP), the acoustic model, pronunciation lexicon, and language model each play distinct roles in evaluating how well a speaker’s utterance matches the expected pronunciation of words in a given language.
There are 3 main parts of the GOP Speechocean recipe from Kaldi.
Acoustic Model: The acoustic model is trained to recognize the various sounds (phonemes) that make up speech. It maps the raw audio features to phonetic units. In the context of GOP, the acoustic model evaluates how closely the sounds in the speaker’s utterance match the expected phonemes of the correct pronunciation. The model’s confidence in phoneme predictions plays a key role in calculating the GOP score.
Pronunciation Lexicon: The pronunciation lexicon provides the expected phonetic transcriptions of words. It is a reference for how words should be pronounced in terms of phonemes. When calculating GOP, the system uses the lexicon to determine the target pronunciation of words or phrases being evaluated. The comparison between this target pronunciation and the spoken pronunciation (as interpreted by the acoustic model) is fundamental to assessing pronunciation quality.
prepare_lang.sh script is used to prepare lexicon and language-specific data files. It includes creating a lexicon.txt file that contains word-to-phone mapping (eg. Hello -> HH EH L OW)
Language Model: While the language model is primarily used to predict the likelihood of word sequences in speech recognition, its role in GOP can be indirect but important. It can help disambiguate phonetically similar words or provide context that makes certain pronunciations more likely than others, thus influencing the assessment of pronunciation quality. The language model can also ensure that the phoneme sequences being evaluated are within plausible linguistic constructs, which can affect the interpretation of pronunciation accuracy.
Training Process
Preparation of Resources
We gathered a phonetically rich and transcribed speech corpus. Then, set up a pronunciation dictionary (lexicon), language models, and necessary configuration files.
Feature Extraction
Extracted acoustic features from the speech corpus. Commonly used features include MFCCs (Mel-Frequency Cepstral Coefficients) or FBANK (Filterbank Energies).
Training Acoustic Models
We then used the extracted features and transcriptions to train acoustic models. The models learn the relationship between the acoustic features and the phonetic units or words.
Training starts with building a simple model:
Monophone Models: These models recognize phonemes without considering context (neighboring phonemes). They are simpler and less accurate but provide a good starting point. Kaldi’s train_mono.sh script is used to perform the monophone training.
Triphone Models: These models consider the context of phonemes (typically the immediate previous and next phonemes). They are more complex and capture more details about speech patterns. Kaldi’s train_deltas.sh script is used to perform the triphone training.
Refinement: Once triphone models are trained, Kaldi’s train_SAT.sh script is used to refine the model to handle different speakers. SAT stands for Speaker Adaptive Training.
Alignment
Performed forced alignment using the trained acoustic models to align the phonetic transcription with the acoustic features. This step is crucial for GOP as it determines how well the predicted phonemes match the phonemes spoken in the audio.
Kaldi provides align_si.sh script just for this purpose!
The script uses the transcriptions and a lexicon (which maps words to their phonetic representations) to compile training graphs. These graphs represent how words (and their phonetic components) can transition during speech according to the language model.
The script performs the alignment task using the training graphs, the existing acoustic model, and the normalized features. This involves determining the most likely sequence of states (which correspond to phonemes or groups of phonemes) that the acoustic model believes were spoken in each training utterance.
GOP Calculation
The Goodness of Pronunciation score is calculated based on the likelihoods produced by the acoustic model during alignment. GOP is a log-likelihood ratio for each phoneme, normalized by the phoneme duration. It indicates how well the phoneme matches the expected model of that phoneme.
GOP is calculated using the compute-gop script of Kaldi. The steps include:
Compute Posteriors: It first computes the posterior probabilities of different phoneme sequences given the acoustic model and the observed features.
Calculate Log-Likelihoods: The script computes the log-likelihoods for each phoneme occurring at each time frame.
Evaluate Pronunciation: GOP is calculated by comparing the log-likelihood of the most likely phoneme sequence (as per the hypothesis) to alternative phoneme sequences. To avoid bias toward longer phonemes, the score is normalized by the duration of the phoneme.
Pronunciation Profiling
The GOP scores can be used to profile the speaker’s pronunciation. Low scores indicate areas where the speaker’s pronunciation deviates from the expected model.
Model Refinement
Based on the GOP scores, we identified the need for additional training data in areas where the pronunciation model is weak. Additional training and refinement of models may occur iteratively.
Application of GOP
Once the system is well-calibrated, GOP scores can be applied in various ways, such as in language learning applications to provide feedback on pronunciation, in speech recognition systems to improve robustness, or in speaker assessment and training tools.
If you are interested in the codebase, check out our GitHub repository
Model Evaluation
A critical phase of our implementation process was rigorous system testing and evaluation. We assessed the model’s performance using Word Error Rate (WER), a common metric in speech recognition that helped us understand how often the model incorrectly predicted phonemes. WER is a critical metric in the evaluation of Automatic Speech Recognition (ASR) systems, serving as a quantifiable measure of transcription accuracy. It is calculated by comparing the ASR system’s output against a reference transcription, taking into account the number of substitutions, deletions, and insertions needed to match the system’s output to the reference.
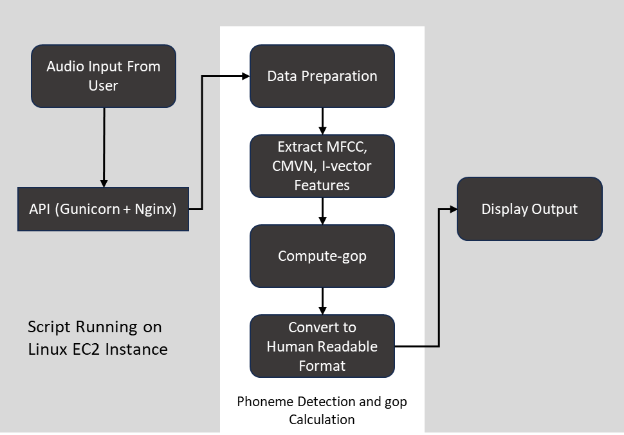
Measurable Impact: Enhancing User Experience and Engagement
The deployment of this phoneme-level pronunciation assessment tool has had a profound impact on the platform’s user engagement metrics. We observed a 12% increase in user engagement, a testament to the enriched learning experience provided by our solution. Furthermore, the platform saw an 8% rise in user retention, indicating that users found the tool engaging and effective in improving their skills. Perhaps most telling was the 10% increase in user referrals and testimonials, a clear indicator of the tool’s impact on users’ language learning journeys and its contribution to positive word-of-mouth for the platform.
Conclusion
Our comprehensive approach to enhancing phoneme detection in language learning platforms has set a new standard in pronunciation training. We have crafted a system that improves pronunciation accuracy and enriches the language learning experience by utilizing advanced technological solutions like the Kaldi ASR toolkit. This project exemplifies our commitment to harnessing advanced technology in addressing educational challenges, contributing significantly to the advancement of language learning methodologies.
Elevate your projects with our expertise in cutting-edge technology and innovation. Whether it’s advancing language learning tools or pioneering in new tech frontiers, our team is ready to collaborate and drive success. Join us in shaping the future—explore our services, and let’s create something remarkable together. Connect with us today and take the first step towards transforming your ideas into reality.
5 Reasons Why Data Analytics is Important for Your Business
5 Reasons Why Data Analytics is Important for Your Business
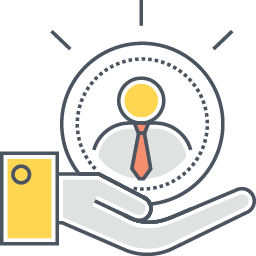
01 Improve Customer Insights
Identify customer’s key problem areas to identify profitable segments and improve customer service through your products and services.
Gatorade was able to achieve 15% growth in a single year through customer insights. (Source: Prophet- Gatorade Case Study)
02 Reduce Costs
Set up efficient processes, ensure optimum resource utilization and introduce flexibility to address market trends.
Carlsberg discovered that giving customers magnetic cards and allowing them to self-pour their beer; resulted in 30% boost in beer consumption and reduced costs as customers moved from fixed price to fixed quantity model. (Source: SAP HANA Blog-Using Big Data to Brew Profits One Pint at a Time)
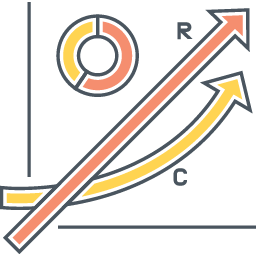
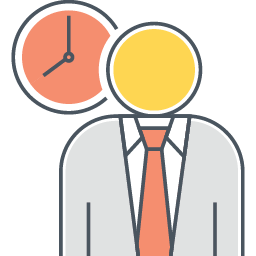
03 Save Valuable Time
Utilize the ability to collect, structure and analyze the data from every aspect of your business at a rapid pace.
Wal-Mart was able to boost revenues through sales of strawberry pop-tarts and beer before hurricane Frances with the help of predictive analytics. (Source: NYT- What Wal-Mart Knows About Customers’ Habits)
04 Propel Decision Making
Access high-quality insights in order to adjust to real-time scenarios and make simultaneous data driven decisions.
According to OKCupid founder, people of the United States hit it off but lose track of their romantic connections mostly in Wal-Mart stores! Now that’s some decision to make! (Source: Dataclysm: Who We Are When We Think No One’s Looking; and Datafloq- 4 Surprising Discoveries from Big Data Insights)
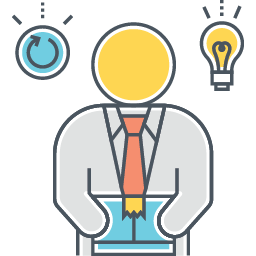
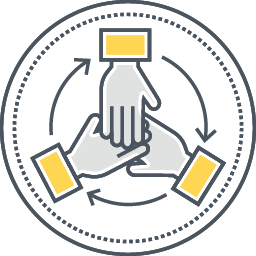
05 Collaboration on-the-go
Access business intelligence insights from the device of your choice whenever you want and wherever you are. Enable your team to gain competitive advantage through data.
TotallyMoney is taking calculated risks and delivering value to their customers before their competition by building a data-driven culture! (Source: TotallyMoney Blog- Building a Data-Driven Culture)
Rudder Analytics Receives a Clutch Leader Award for 2019!
Since 2015, Rudder Analytics has been working hard to steer businesses in the right direction through end to end data analytics services including Data ETL, Machine Learning, Business Intelligence and Data Visualization services.
According to a Forbes article, a CMO Survey concluded that companies are projected to “increase the marketing analytics portion of their marketing budgets by 60% in the next three years.” Marketing analytics insights are what keeps our gears turning day in and day out. Rudder Analytics will be right there alongside the boom, which makes us even more excited to announce that this month, we have received a Clutch Leader Award as one of the leading marketing analytics companies on their site!
Clutch is a B2B ratings and reviews site that works to connect businesses together worldwide. Their research centers around a company’s market presence, work quality, and client experience. Clutch’s team of analysts interview prior clients and publish the feedback on the company’s Clutch profile to help companies gain credibility and success in the eyes of others.
We take this opportunity to thank our clients for their continued trust in our ability to deliver and for their support throughout these years of excellence!
Furthermore, we have received recognition on Clutch’s sister websites, The Manifest and Visual Objects. The former is a business news and how-to website that compiles and analyzes practical business wisdom and the latter, a platform for companies to showcase their portfolios to potential clients. We are thrilled to be listed in their big data companies and developers directories.
Rudderite featured on Microsoft Power BI Data Stories Gallery
Congratulations to our data-storyteller Ketan Deshpande for getting featured on Microsoft Power BI Data Stories Gallery and earning much-deserved appreciation from the Microsoft Power BI community.
These entries were judged by the Microsoft Data Journalism Team, and the featured stories were selected for telling a compelling story, being original and creative, and effectively using Microsoft Power BI.
Rudder Analytics ranked highly in Top Indian IT Services
In this age of data, all business decisions are backed by analyzing data scientifically and Rudder Analytics has been helping businesses in all aspects of analytics.
It has been one and a half year since we started operations and we have already received rave reviews for our quality services. Rudder Analytics was recently ranked highly in the press release of IT Services review by Clutch.co.
Clutch, an independent ratings and reviews site based in Washington, D.C. that covers top companies in IT services, awarded us at Rudder Analytics earlier this month a distinction as being one of the top companies in its research and thus a place in its India IT Services Leaders Matrix.
Our focus on niche categories of IT services from our basket of visual analytics with Tableau, Qliksense, Power BI etc., data analytics and statistical modeling services, along with strong praise from past clients earned us the compliment. For example, consider the all 5-star rating from one of our clients and their report that: “Rudder Analytics was able to provide that extra kick.”
We are really pleased to receive any feedback from our clients but especially happy to see a very satisfied customer – it means we have done our job and done it well. Thank you to everyone who has participated in the review process. Please take a minute to see our Rudder Analytics coverage in full.
Rudder Analytics got featured on CNBC as one of the leading IT services firms in India
Rudder Analytics has been featured on CNBC as one of the leading IT services firms in India and this is what the analysts had to say about us:
“These companies are the finest examples of outsourced IT services firms in India based on our current book of research,” said Clutch analyst Clayton Kenerson. “All of these companies should be proud of their clients’ reviews – the proof of their professionalism and technical prowess that lends them hard-earned distinction in an otherwise crowded market.”
http://www.cnbc.com/2016/09/08/pr-newswire-clutch-recognizes-leading-it-services-firms-in-india.html