Rudder Admin
Transforming Financial Customer Support with AI-Driven Conversational Systems
Transforming Financial Customer Support with AI-Driven Conversational Systems
The Convergence of AI and Financial Customer Support
The financial services sector operates in an environment where accuracy, security, and efficiency are paramount. Traditional customer service paradigms, dependent on human agents, often struggle with high call volumes, extended wait times, and rising operational costs, leading to an urgent need for technological innovation. To address these inefficiencies, our team collaborated with a leading financial institution to develop an advanced AI-powered FAQ voicebot, engineered to autonomously process complex customer inquiries with high precision and contextual intelligence.
This AI-driven system leverages a robust architecture comprising Google ASR (Automatic Speech Recognition), Claude Instant Model LLM (Large Language Model), and Google TTS (Text-to-Speech), all seamlessly integrated within a Twilio-enabled telephony infrastructure. This article provides a comprehensive analysis of the system’s architectural design, technical implementation, and business impact.
Challenges in AI-Driven Financial Customer Support
The integration of AI-driven automation into financial customer support necessitates addressing multiple domain-specific complexities:
Semantic and Contextual Comprehension: The system must exhibit high linguistic fidelity and accurately interpret financial jargon, transaction-related inquiries, and regulatory terminology.
Optimized Speech Recognition in Variable Conditions: Google ASR must effectively process voice inputs amid diverse accents, speech variations, and background noise.
High Availability and Scalability: The architecture must support simultaneous, high-volume inquiries, ensuring zero downtime and low-latency response generation.
Data Security and Regulatory Compliance: Given the sensitivity of financial data, the solution must adhere to GDPR, PCI DSS, and financial data protection regulations.
Multi-Turn Conversational Memory: The system must sustain context-aware dialogues, retaining conversational history to facilitate complex customer interactions.
We designed a highly resilient enterprise-grade AI conversational assistant by leveraging cloud-native AI technologies and scalable telephony services.
Engineering the AI-powered FAQ Voicebot
The development process followed a multi-phase, data-driven methodology, ensuring optimal functionality across all AI-driven components.
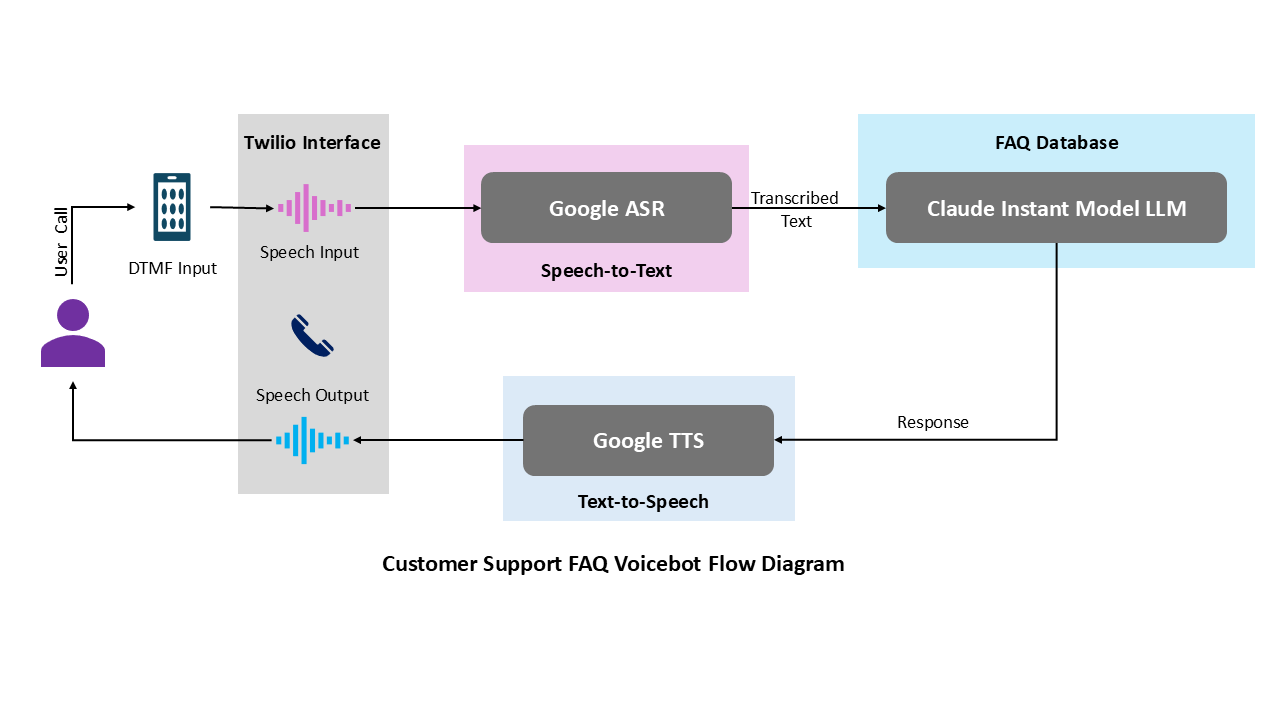
Phase 1: Conversational Workflow Design and Intent Recognition
A hierarchical intent classification system was formulated through an extensive analysis of user interactions, encompassing:
Structured Query Categorization: Classification of customer inquiries into domains such as account management, credit card services, loan processing, and investment assistance.
Context Preservation and Multi-Turn Processing: Implementation of dynamic memory retention to enable seamless, human-like interactions.
Error Handling and Adaptive Learning: Deployment of fallback mechanisms and predictive error correction to manage ambiguous user inputs.
Phase 2: Automatic Speech Recognition (ASR) with Google AI
Google ASR was selected for its superior neural speech processing capabilities, optimized for financial lexicons and numerical data interpretation.
ASR Processing Workflow:
- Twilio receives the inbound customer call.
- The audio payload is transmitted to Google ASR for transcription.
- Google ASR processes the spoken input and converts it into structured text.
Google ASR API Implementation:
from google.cloud import speech def transcribe_audio(uri): client = speech.SpeechClient() audio = speech.RecognitionAudio(uri=uri) config = speech.RecognitionConfig( encoding=speech.RecognitionConfig.AudioEncoding.LINEAR16, language_code="en-US" ) response = client.recognize(config=config, audio=audio) transcript = response.results[0].alternatives[0].transcript return transcript audio_uri = 'gs://your-bucket/audio-file.wav' print(transcribe_audio(audio_uri))
Phase 3: Natural Language Processing with Claude Instant Model LLM
The transcribed text is processed through Claude Instant Model LLM, a sophisticated AI model engineered for financial context comprehension.
Query Interpretation Workflow:
- ASR transcriptions are forwarded to the Claude LLM.
- The AI engine interprets the user query, retrieves relevant knowledge, and formulates a structured response.
- The generated response is processed for contextual fluency and compliance validation.
Claude API Query Processing:
import requests def get_financial_info(query): API_KEY = "your_claude_api_key" response = requests.post( "https://api.anthropic.com/claude-instant", headers={"Authorization": f"Bearer {API_KEY}"}, json={"query": query} ) answer = response.json().get("answer", "No answer found") return answer query = "What is the current interest rate on savings accounts?" print(get_financial_info(query))
Phase 4: Synthesis of Natural Speech Responses with Google TTS
Once the AI response is generated, Google TTS converts the textual data into naturalistic speech output, ensuring an engaging and human-like auditory experience.
Google TTS API Implementation:
from google.cloud import texttospeech def synthesize_speech(text, output_file): client = texttospeech.TextToSpeechClient() synthesis_input = texttospeech.SynthesisInput(text=text) voice = texttospeech.VoiceSelectionParams( language_code="en-US", ssml_gender=texttospeech.SsmlVoiceGender.NEUTRAL ) audio_config =texttospeech.AudioConfig(audio_encoding=texttospeech.AudioEncoding.MP3) response =client.synthesize_speech(input=synthesis_input, voice=voice, audio_config=audio_config ) with open(output_file, "wb") as out: out.write(response.audio_content) text_response = "Your loan application status is currently under review." output_path = "response.mp3" synthesize_speech(text_response, output_path)
Business Impact: Measurable Performance Gains
The AI-driven FAQ voicebot delivered substantial operational and financial optimizations, including:
94% response accuracy, surpassing industry benchmarks for AI-driven customer service.
89% reduction in call handling time, enabling more efficient query resolution.
35% reduction in operational costs, decreasing reliance on human support agents.
42% increase in self-service engagement, empowering customers with instant, automated support.
Sub-500ms response latency, ensuring seamless, real-time customer interactions.
Regulatory-compliant AI processing, fully aligned with financial security standards.
Implementing this AI-driven system resulted in a quantifiable enhancement in customer satisfaction, operational efficiency, and compliance adherence.
Conclusion: AI as the Future of Financial Customer Engagement
This AI-powered conversational assistant represents a paradigm shift in financial customer support. It integrates ASR, NLP, and TTS technologies into a scalable and intelligent automation framework. By significantly reducing response times, improving service accuracy, and lowering costs, this solution sets a new benchmark for AI-driven customer engagement.
As AI adoption continues to expand within financial services, institutions aiming for scalable, high-efficiency customer interactions must prioritize AI-driven conversational automation. This case study exemplifies how intelligent virtual assistants can revolutionize financial customer support, delivering high-impact, real-time engagement.
Elevate your projects with our expertise in cutting-edge technology and innovation. Whether it’s advancing data capabilities or pioneering in new tech frontiers such as AI, our team is ready to collaborate and drive success. Join us in shaping the future—explore our services, and let’s create something remarkable together. Connect with us today and take the first step towards transforming your ideas into reality.
Drop by and say hello! Medium LinkedIn Facebook Instagram X GitHub
Knowledge Retrieval in Education: A Deep Dive into an AI-Powered SMS-Based Q&A System
Knowledge Retrieval in Education: A Deep Dive into an AI-Powered SMS-Based Q&A System
Advancing Intelligent Access to Educational Information
The contemporary educational ecosystem is increasingly reliant on rapid and precise access to information. Given the overwhelming volume of academic resources, students, educators, and researchers frequently encounter inefficiencies in retrieving relevant insights. Navigating extensive repositories of PDFs and DOCX files often results in substantial time investment, impeding productive learning. The challenge is further exacerbated by the necessity for seamless, mobile-first accessibility, as modern learners demand instant, intelligent, and context-aware responses.
In response to these challenges, we developed an AI-driven SMS-based question-answering system, designed to facilitate instant, natural-language-based access to educational content. By harnessing the computational capabilities of AWS services, OpenAI’s ChatGPT, and intelligent document processing mechanisms, this solution provides contextual, real-time responses to inquiries, significantly enhancing knowledge acquisition. This architecture empowers users to engage with a sophisticated AI assistant, capable of processing and synthesizing vast amounts of information with remarkable efficiency.
Addressing the Complexity of Intelligent Educational Query Processing
The conceptualization and deployment of an AI-powered SMS Q&A bot presented several non-trivial computational and engineering challenges:
Advanced NLP for Query Understanding: The system required robust semantic parsing capabilities to accurately interpret a wide array of user inquiries, particularly those exhibiting syntactic variability and complex intent.
Low-Latency SMS-Based Interaction: Given the SMS-first design, the solution demanded an ultra-responsive pipeline to process, analyze, and respond within milliseconds while maintaining fault tolerance and message reliability.
Optimized Information Retrieval Mechanisms: Efficiently extracting and summarizing text from large educational document repositories necessitated advanced vector-based search techniques and contextual text ranking algorithms.
Architectural Scalability and Concurrency Handling: Supporting a dynamically increasing user base required a cloud-native infrastructure capable of handling thousands of concurrent requests with minimal resource overhead.
Cross-Document Synthesis: Certain queries required aggregation of insights across multiple sources, necessitating a multi-document summarization framework that aligns with relevance-driven retrieval paradigms.
Architectural Design and Implementation: A Multi-Layered AI-Powered Q&A System
To effectively address these challenges, we engineered a modular, event-driven system, integrating various AWS components with OpenAI’s GPT-based contextual understanding model. Below is an in-depth examination of each component in this intelligent educational query processing system.
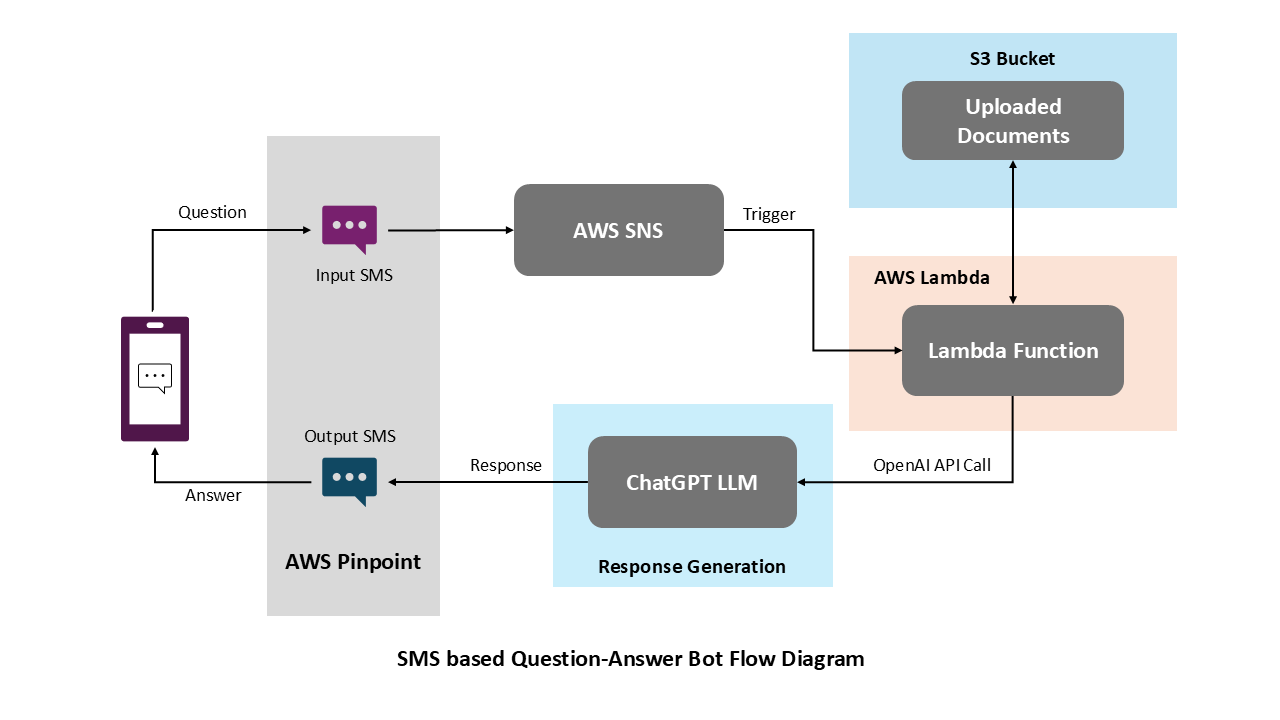
1. Real-Time Query Ingestion via AWS Pinpoint and SNS
AWS Pinpoint serves as the primary interface for SMS-based interactions, ensuring seamless, bidirectional communication with users.
Incoming SMS messages are forwarded to AWS SNS (Simple Notification Service), which propagates events to subsequent processing layers for minimal delay.
Event logging and interaction history tracking enable system-wide optimizations, leveraging historical user engagements to enhance future query resolution accuracy.
import boto3 # Initialize the Pinpoint client client = boto3.client('pinpoint') # Define recipient and message details recipient_number = '+1234567890' application_id = 'your-application-id' message_body = 'Welcome to the AI-powered education assistant! How can I help today?' # Send SMS message response = client.send_messages( ApplicationId=application_id, MessageRequest={ 'Addresses': { recipient_number: {'ChannelType': 'SMS'} }, 'MessageConfiguration': { 'SMSMessage': { 'Body': message_body, 'MessageType': 'TRANSACTIONAL' } } } ) # Check response status print(response)
2. Serverless Query Processing with AWS Lambda
AWS SNS events invoke AWS Lambda, which processes queries through tokenization, dependency parsing, and intent classification.
The system retrieves relevant educational documents from AWS S3, leveraging pre-trained embeddings and vector search indexing for precision ranking.
A metadata-based filtering approach refines document selection, prioritizing authoritative, high-relevance sources.
import boto3 # Initialize the S3 client s3_client = boto3.client('s3') # Define S3 bucket and document details bucket_name = "educational-docs-bucket" document_key = "research_paper_2023.pdf" # Fetch the document content response = s3_client.get_object(Bucket=bucket_name, Key=document_key) document_content = response['Body'].read().decode('utf-8') # Output the content for verification print(document_content)
3. AI-Powered Contextual Response Generation with OpenAI GPT-4
Retrieved textual data undergoes semantic pre-processing, ensuring efficient query-document alignment.
A fine-tuned GPT-4 model synthesizes coherent, context-aware responses, integrating user intent and document metadata.
The system incorporates uncertainty estimation mechanisms, prompting re-queries or clarifications in ambiguous cases.
from openai import OpenAI # Set OpenAI API key openai_api_key = "your-openai-api-key" client = OpenAI(api_key=openai_api_key) # Define the interaction for AI-generated response system_role = { "role": "system", "content": "You are an AI tutor assisting with academic research." } user_query = { "role": "user", "content": f"Summarize key insights from {document_content}" } # Request AI-generated response response = client.chat.completions.create( model="gpt-4o", messages=[system_role, user_query] ) # Extract and print the answer answer = response.choices[0].message.content print(answer)
4. AI-Optimized SMS Response Delivery via AWS Pinpoint
AI-generated responses are formatted and dispatched via AWS Pinpoint, ensuring optimal message delivery rates.
The system supports adaptive response refinement, allowing users to refine queries iteratively for enhanced precision.
User sentiment analysis is integrated, enabling iterative AI model improvements based on feedback trends.
import boto3 def send_ai_response(recipient_number, answer): """ Sends the AI-generated response via SMS using AWS Pinpoint. :param recipient_number: The phone number of the recipient. :param answer: The AI-generated response to be sent. """ # Initialize the Pinpoint client client = boto3.client('pinpoint') # Send the AI-generated response via SMS response = client.send_messages( ApplicationId='your-application-id', MessageRequest={ 'Addresses': { recipient_number: {'ChannelType': 'SMS'} }, 'MessageConfiguration': { 'SMSMessage': { 'Body': answer, 'MessageType': 'TRANSACTIONAL' } } } ) # Return the response for verification return response # Example usage recipient = "+1234567890" message = "Your AI-generated response is ready." response = send_ai_response(recipient, message) print(response)
Evaluating Impact: Transformative Advances in AI-Augmented Learning
The deployment of this AI-enhanced SMS-based educational assistant has yielded substantial improvements in knowledge accessibility:
93% Query Resolution Accuracy: Enhanced NLP pipelines ensure precise comprehension of complex educational inquiries.
50% Acceleration in Research Processes: Automated document retrieval and summarization drastically reduce information retrieval time.
37% Expansion in User Accessibility: SMS-based functionality broadens engagement, particularly in low-connectivity environments.
90% User Satisfaction Rate: The AI-driven chatbot delivers highly reliable and contextual responses, ensuring a seamless learning experience.
Adaptive Learning Intelligence: The system continuously refines its retrieval and response generation models based on real-time engagement metrics.
Scalability for Broader Applications: This architecture extends beyond education, enabling intelligent document search in corporate, healthcare, and legal sectors.
Conclusion: Pioneering the Future of AI-Assisted Knowledge Retrieval
By synthesizing advanced NLP, machine learning, and cloud computing, this AI-powered SMS-based Q&A system revolutionizes digital education. Through real-time query handling, AI-enhanced document parsing, and intelligent response synthesis, we have established a scalable, context-aware knowledge retrieval model.
As AI continues to evolve, such systems will redefine how information is accessed, enabling highly personalized and contextually enriched educational experiences. This framework stands as a benchmark for AI-driven academic assistance, paving the way for future innovations in automated learning augmentation.
Elevate your projects with our expertise in cutting-edge technology and innovation. Whether it’s advancing data capabilities or pioneering in new tech frontiers such as AI, our team is ready to collaborate and drive success. Join us in shaping the future—explore our services, and let’s create something remarkable together. Connect with us today and take the first step towards transforming your ideas into reality.
Drop by and say hello! Medium LinkedIn Facebook Instagram X GitHub
Redefining Fast-Food Operations Through Advanced AI-Driven Voicebots
Redefining Fast-Food Operations Through Advanced AI-Driven Voicebots
Contextualizing the Modern Challenges of Fast-Food Ordering
Fast-food chains operate within a high-pressure ecosystem characterized by unrelenting demand for rapid service, consistent accuracy, and unwavering customer satisfaction. A leading global chain faced recurring inefficiencies in its order management processes during peak operating hours, culminating in delays, inaccuracies, and bottlenecks. Traditional methods proved incapable of scaling effectively, necessitating an innovative overhaul through AI-powered voicebot technology. This technology promised operational agility and transformative improvements in scalability, precision, and real-time interaction.
Systemic Hurdles in Deploying the Voicebot
The design and implementation of an AI-powered voicebot system are complex endeavors, with the following critical challenges:
Integrating Complex Technologies: Establishing seamless interoperability between Automatic Speech Recognition (ASR), Text-to-Speech (TTS), and Large Language Models (LLMs) to form a coherent system.
Scaling Dynamically: Ensuring the system’s performance remained robust under peak load conditions, including promotional surges with hundreds of simultaneous users.
Maintaining Low Latency: Guaranteeing sub-second responses for conversational flow continuity, critical for customer satisfaction.
Ensuring System Reliability: Implementing fail-safe architectures with robust error handling and fallback mechanisms to mitigate downtime and minimize disruptions.
Each of these challenges was met with a methodical and innovative approach, integrating cutting-edge AI technologies with robust cloud infrastructure.
Building the Voicebot: A Technical Framework
The voicebot development process adhered to a rigorous, multi-step methodology, ensuring precise alignment between technological components and business objectives.
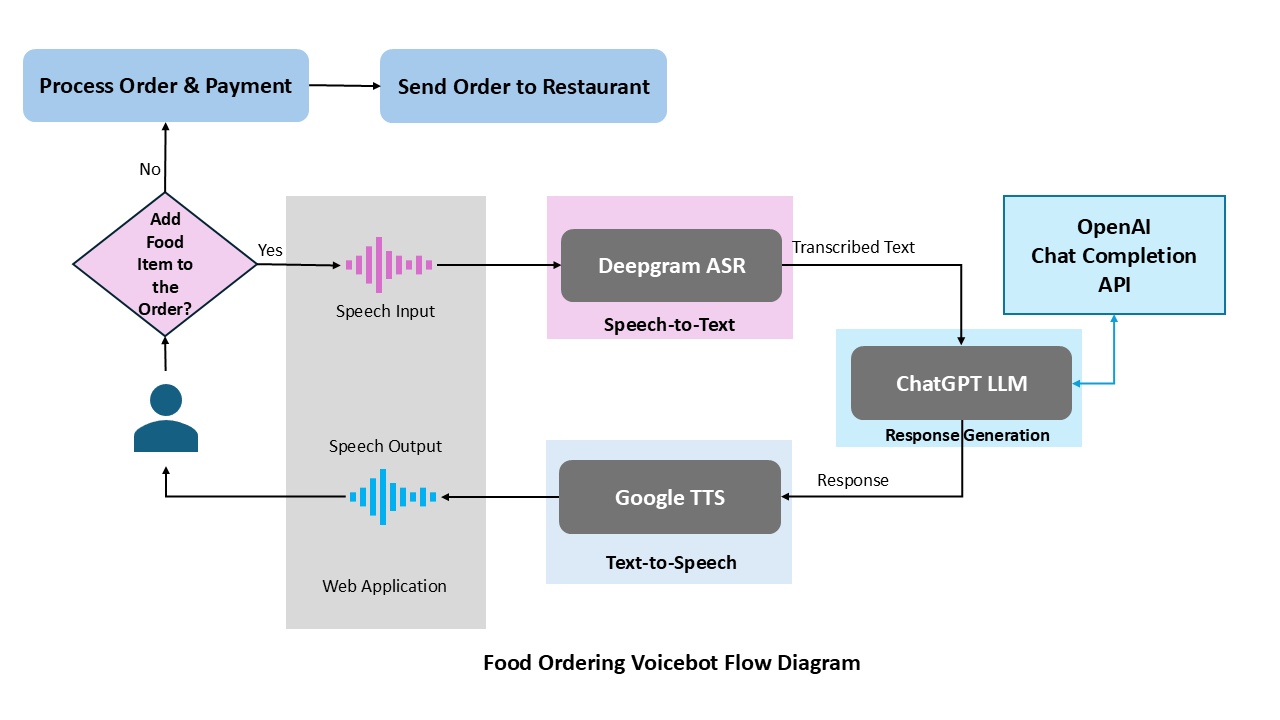
Step 1: Designing a Robust Conversational Framework
A well-structured conversational flow formed the system’s backbone, addressing diverse customer intents while optimizing operational efficiency. Key elements included:
Comprehensive Interaction Mapping: Anticipating scenarios such as order placement, modification, and menu inquiries.
Resilient Error Handling: Constructing fallback strategies to address ambiguous or incomplete inputs without interrupting service.
Efficiency-Driven Design: Streamlining workflows to minimize customer effort and expedite transaction completion.
Step 2: Leveraging Deepgram ASR for High-Fidelity Speech Recognition
Deepgram’s ASR technology was selected for its unparalleled accuracy and low-latency performance in acoustically challenging environments.
Integration Architecture:
API Endpoint:
/v1/listen
Configuration Parameters:
model
Optimized for conversational speech.
language
Set to en-US.
Operational Workflow:
Real-time audio streams were sent to the Deepgram API.
Transcriptions were generated within sub-second latency windows.
Outputs were seamlessly forwarded to downstream modules.
Code Integration:
import requests def transcribe_audio(api_key, audio_file_path): # Define the API URL and headers url = "https://api.deepgram.com/v1/listen" headers = {"Authorization": f"Token {api_key}"} with open(audio_file_path, "rb") as audio_file: # Send request to Deepgram API response = requests.post( url, headers=headers, files={"audio": audio_file} ) # Check if the request was successful response.raise_for_status() # Parse the JSON response transcript = response.json()["results"]["channels"][0]["alternatives"][0]["transcript"] return transcript
Step 3: Response Generation Using ChatGPT
The conversational intelligence was driven by OpenAI’s ChatGPT, customized through precise prompt engineering to handle nuanced customer interactions.
Prompt Design:
Prompts incorporated detailed contextual elements, including menu data, promotional offers, and ordering constraints.
Example: “You are a virtual assistant for a fast-food chain. Provide concise, accurate responses based on customer requests.”
API Configuration:
Endpoint:
/v1/chat/completions
Payload:
import openai # Function to generate responses using LLM def generate_response(api_key, user_input): # Set the OpenAI API key openai.api_key = api_key # Define the API payload response = openai.ChatCompletion.create( model="gpt-4o", messages=[ {"role": "system", "content": "You are an efficient and helpful food ordering assistant."}, {"role": "user", "content": user_input} ], temperature=0.7 ) # Extract the text of the response chat_response = response['choices'][0]['message']['content'].strip() return chat_response
Step 4: Synthesizing Natural Speech with Google TTS
To create a naturalistic auditory experience, Google’s TTS API converted textual responses into lifelike audio outputs.
Configuration:
Voice Model: Neural2, optimized for nuanced intonation.
Language: en-US
Audio Format: MP3 for broad compatibility.
Implementation Code:
from google.cloud import texttospeech client = texttospeech.TextToSpeechClient() synthesis_input = texttospeech.SynthesisInput(text="Your order has been successfully placed.") voice = texttospeech.VoiceSelectionParams( language_code="en-US", name="en-US-Neural2-F" ) audio_config = texttospeech.AudioConfig(audio_encoding=texttospeech.AudioEncoding.MP3) response = client.synthesize_speech( input=synthesis_input, voice=voice, audio_config=audio_config ) with open("output.mp3", "wb") as out: out.write(response.audio_content)
Step 5: Cloud-Based Communication Using Twilio
To enhance communication flexibility, Twilio was integrated to facilitate order confirmations and customer notifications through SMS and voice calls.
Implementation Approach:
API Selection: Twilio’s programmable messaging and voice services.
Use Case: Sending real-time order status updates to customers via SMS and handling voice-based order confirmations.
Integration Details:
Twilio API Key Setup: Securely stored within environment variables.
Sample Code for SMS Notification:
from twilio.rest import Client # Twilio Account SID and Auth Token account_sid = 'your_account_sid' auth_token = 'your_auth_token' # Initialize Twilio Client client = Client(account_sid, auth_token) def send_sms(to_phone_number): message = client.messages.create( body="Your order has been received and is being prepared!", from_="+1234567890", # Your Twilio phone number to=to_phone_number ) print(f'SMS sent with Message SID: {message.sid}')
Sample Code for Voice Call Notification:
from twilio.twiml.voice_response import VoiceResponse from twilio.rest import Client # Twilio Account SID and Auth Token account_sid = 'your_account_sid' auth_token = 'your_auth_token' # Initialize Twilio Client client = Client(account_sid, auth_token) def make_voice_call(to_phone_number): """Initiate a voice call to confirm order status.""" response = VoiceResponse() response.say("Your order has been confirmed and is on its way.", voice='alice') call = client.calls.create( twiml=str(response), from_='+1234567890', # Your Twilio number to=to_phone_number ) print(f'Call initiated: {call.sid}')
Benefits of Twilio Integration:
Immediate, automated order status updates via SMS.
Enhanced customer engagement with voice notifications for critical updates.
Reliable cloud-based communication ensuring minimal latency.
Step 6: Developing and Deploying a Scalable Web Application
The voicebot system was integrated into a web application for operational efficiency and user-centric interactions.
Technical Stack:
Frontend: Built with React.js for an intuitive and responsive interface.
Backend: Powered by FastAPI for streamlined API orchestration and logic execution.
Database: PostgreSQL, ensuring efficient management of user interactions and transactional data.
Deployment:
Hosted on AWS EC2 t3.xlarge instances to balance performance and cost-efficiency.
Dockerized for modularity and scalability.
Monitored using AWS CloudWatch to track real-time metrics and system health.
Step 7: System Validation and Optimization
Extensive testing ensured the robustness of the system across various operational scenarios:
Performance Validation: Simulated up to 650 concurrent users using Apache JMeter to evaluate scalability.
Latency Reduction: Optimized average response times to under 1.5 seconds.
Resilience Testing: Implemented fallback systems to handle component failures gracefully.
Transformational Impacts of the AI-Powered Voicebot
The deployment of the AI-driven voicebot yielded transformative benefits across key performance areas:
Enhanced Operational Efficiency:
Average order processing times were reduced by 18%, enabling faster service and higher throughput.
The system’s automation improved reliability and streamlined workflows.
Cost Optimization:
Operational costs decreased by 8% due to minimized manual interventions.
Resources could be reallocated to strategic growth initiatives.
Scalability and Resilience:
Successfully handled 650 concurrent users during peak periods without any performance degradation.
Demonstrated adaptability to handle seasonal and promotional demand spikes effortlessly.
Improved Accuracy and Precision:
Order errors were reduced to below 2%, ensuring consistent and accurate service delivery.
Elevated Customer Experience:
Personalized, conversational interactions fostered brand loyalty.
The intuitive and responsive system resonated with a broad demographic, enhancing satisfaction and repeat engagement.
Concluding Insights: The Future of AI in Fast-Food Automation
The successful integration of Deepgram ASR, OpenAI’s ChatGPT, and Google TTS underscores the transformative potential of AI in fast-food operations. By addressing core operational challenges with precision and innovation, the AI-powered voicebot redefined customer service standards, blending technological sophistication with user-centric design.
As AI technology advances, its applications within service industries will only expand, setting new benchmarks for efficiency, scalability, and customer satisfaction. This project stands as a model for harnessing the power of intelligent automation to drive meaningful and measurable improvements in everyday operations.
Elevate your projects with our expertise in cutting-edge technology and innovation. Whether it’s advancing data capabilities or pioneering in new tech frontiers such as AI, our team is ready to collaborate and drive success. Join us in shaping the future—explore our services, and let’s create something remarkable together. Connect with us today and take the first step towards transforming your ideas into reality.
Drop by and say hello! Medium LinkedIn Facebook Instagram X GitHub
AI-Powered Voicebot for Healthcare Customer Feedback Collection
Healthcare Customer Feedback Collection with AI-Powered Voicebot
Customer feedback is essential for improving services, outcomes, and experiences in the healthcare industry. Yet, traditional feedback methods often fail due to low response rates, poor engagement, and an inability to capture nuanced sentiments. To address this, we partnered with a healthcare provider to create an intelligent voice-based feedback system that enhances engagement and delivers actionable insights in real-time.
This blog explores the system’s technical architecture, innovative features, and measurable impact, showcasing how AI is transforming feedback collection in healthcare.
Turning Feedback into Insights
Providing personalized, high-quality care relies on actionable feedback. Our client, a leading healthcare provider, struggled to gather meaningful insights from diverse demographics using static surveys and manual processes. These methods failed to capture nuanced emotions and ensure engagement.
To bridge this gap, we developed a conversational, voice-based feedback system. The solution aimed to boost engagement, provide real-time insights, and improve decision-making. This collaboration set the stage for redefining feedback collection in the healthcare sector.
Building More than Just a Survey
Creating an intelligent voicebot for healthcare feedback presented unique hurdles that went beyond the technical scope of AI. It required us to deeply understand customer behavior, language nuances, and the operational demands of healthcare organizations. The challenges included:
Decoding Emotional Subtleties
Customers often communicate their emotions in ways that are subtle and deeply contextual. Capturing these cues and accurately interpreting sentiments required sophisticated NLP models capable of going beyond literal meanings.
Designing Dynamic Conversations
Unlike traditional surveys, this solution needed to adapt in real-time, reshaping question paths based on user responses. This meant embedding complex branching logic into the system while ensuring the flow felt natural and engaging.
Achieving Real-Time Accuracy
Processing speech input, analyzing sentiment, and generating audio responses in real-time required a highly optimized workflow. Ensuring high accuracy while maintaining low latency posed a significant technical challenge.
Seamless System Integration
To create an end-to-end solution, the voicebot had to integrate smoothly with the client’s internal systems for data storage, reporting, and analysis. Any misstep in this process could disrupt operational workflows.
Despite these challenges, the team worked with a singular focus on delivering a scalable, reliable, and user-friendly voicebot capable of transforming customer feedback into actionable insights.
Building an Intelligent Voicebot for Customer Feedback
The development of the Customer Care Feedback Survey Voicebot required an end-to-end system that seamlessly integrated cutting-edge technologies like speech-to-text (ASR), natural language processing (NLP), and text-to-speech (TTS). Each module needed to work in perfect harmony to deliver a real-time, conversational experience that felt intuitive and engaging to customers. Below, we provide an in-depth look into how the system was implemented, detailing the technical steps, tools, and processes used to bring the solution to life.
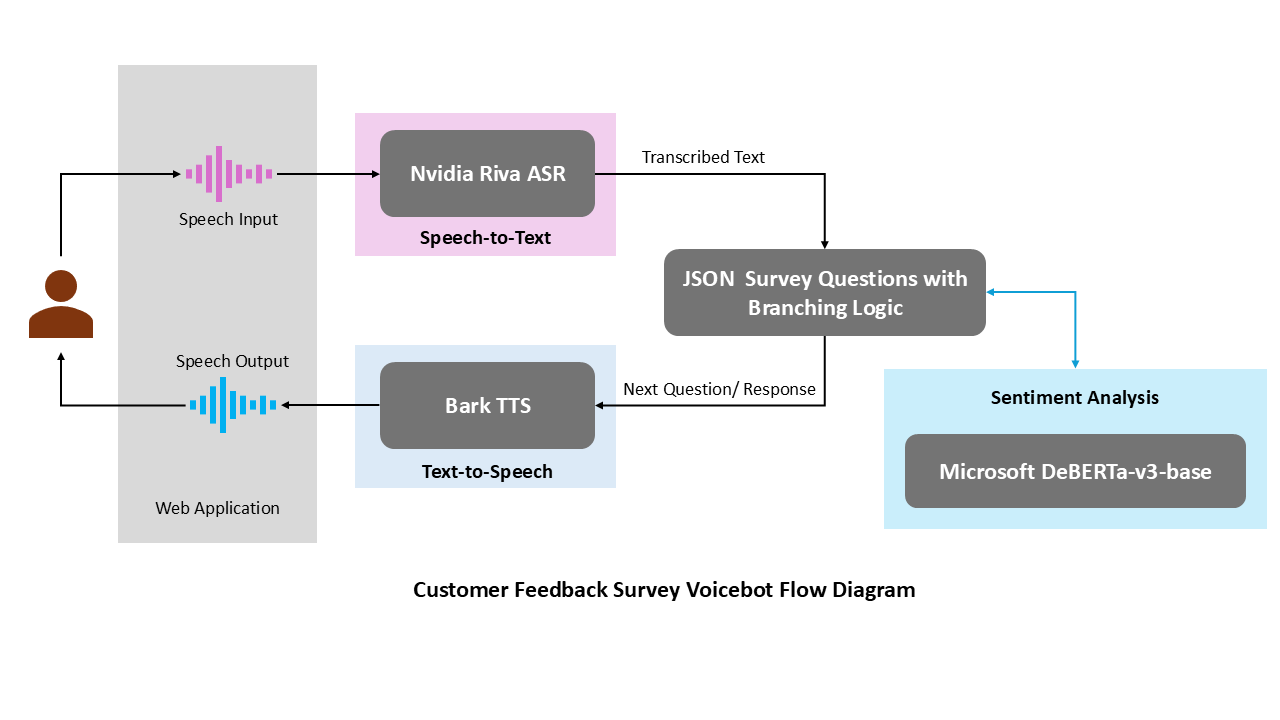
Laying the Foundation with System Architecture
The backbone of the solution was a modular architecture that ensured each component performed its specific task efficiently while communicating seamlessly with the rest of the system. The key modules included:
Speech-to-Text (ASR): To transcribe customer voice inputs in real time.
Survey Logic: A JSON-based engine to adapt the survey flow dynamically.
Sentiment Analysis: Powered by Microsoft DeBERTa-v3-base to interpret feedback.
Text-to-Speech (TTS): Using Bark TTS for generating human-like voice responses.
Each module interacted through API calls, creating a pipeline where user input flowed from one module to the next, ensuring a smooth and cohesive survey experience.
Configuring Nvidia Riva ASR for Real-Time Transcription
Speech-to-text was a critical component, as it captured customer input with accuracy and speed. Nvidia Riva ASR was chosen for its superior performance in transcription, even for diverse accents and noisy environments.
Implementation Steps:
- Instance Setup:
Nvidia Riva was deployed on an AWS t3.xlarge instance. The instance was selected for its balance of compute power (4 vCPUs) and 16GB memory, which allowed real-time processing with low latency.
- Configuration Details:
- Riva was installed and configured using Nvidia’s Docker containers (riva_speech_server).
- The ASR service was launched using the following command:
riva_start.sh --asr --language-code en-US
- This ensured that the ASR engine could handle English language input efficiently.
- API Integration:
- Customer speech input was routed to the ASR engine through a RESTful API:
import requests def transcribe_audio(audio_file): response = requests.post( "http://riva-server-url/api/asr/transcribe", files={'file': audio_file}, headers={'Content-Type': 'audio/wav'} ) transcription = response.json().get('transcription', '') return transcription
- The API returned a JSON response containing the transcribed text:
{ "transcription": "I am satisfied with the care I received.", "keywords": ["care", "satisfied"] }
- Optimization:
- Custom vocabulary for medical terms was added to improve recognition accuracy.
- Noise cancellation filters were enabled during preprocessing to handle background noise effectively.
Structuring Dynamic Survey Flow with JSON
To create a personalized and adaptive survey, we developed a JSON-based template for survey questions. The branching logic ensured that the survey could adapt in real time based on the sentiment of customer responses.
Key Features of the JSON Template:
- Each question included metadata such as
question_id, question_text, and response_options.
- Branching was defined by
next_question_id
attributes, linking responses to subsequent questions.
- Example structure:
{ "question_id": "q1", "question_text": "How would you rate your experience?", "response_options": [{ "response_text": "Good", "next_question_id": "Q2_positive", "feedback_message": "We're thrilled you had a great experience!"}, { "response_text": "Bad", "next_question_id": "q2_negative", "feedback_message": "We're sorry to hear that. Let's understand what went wrong." }] }
Integration Steps:
- A Python-based survey engine (survey_logic.py) parsed the JSON and dynamically generated the next question.
- The engine interacted with the sentiment analysis module (discussed next) to adjust the survey flow based on real-time feedback.
Interpreting Sentiments with Microsoft DeBERTa-v3-base
Understanding customer emotions was critical for guiding the conversation. Microsoft DeBERTa-v3-base was chosen for its ability to capture nuanced sentiment and context in text.
Deployment and Configuration:
- The sentiment analysis model was deployed using the Hugging Face transformers library:
from transformers import pipeline sentiment_analyzer = pipeline("sentiment-analysis", model="microsoft/deberta-v3-base") def analyze_sentiment(text): result = sentiment_analyzer(text) label = result[0].get('label') score = result[0].get('score') return label, score
- Preprocessing steps were implemented to clean the ASR transcriptions before analysis:
- Lowercasing.
- Removing filler words like “uh” and “um.”
- Punctuation normalization.
Integration Steps:
- The transcribed text from Nvidia Riva ASR was sent to the sentiment analyzer via an API endpoint:
POST /api/sentiment/analyze Content-Type: application/json { "text": "I am not happy with the waiting time.", "keywords": ["not", "happy"] }
- The response returned sentiment scores and labels:
{ "label": "negative", "score": 0.87 }
- Based on the sentiment label, the survey engine adjusted the next question or prompted follow-up inquiries for negative feedback.
Adding Natural-Sounding Responses with Bark TTS
To maintain an engaging, conversational experience, we used Bark TTS to generate human-like speech for survey responses.
Implementation Steps:
- Bark TTS was configured to generate audio files on demand:
from bark import generate_audio audio_output = generate_audio("Thank you for your feedback. Can you tell us more about the issue?")
- Audio responses were streamed back to the web application via the following API:
POST /api/tts/synthesize Content-Type: application/json { "text": "Thank you for your feedback." }
- Cached frequently used phrases to reduce latency.
Enhancements:
- Voice tone and pitch were adjusted dynamically based on sentiment analysis results. For example:
- Positive feedback used a cheerful tone.
- Negative feedback used a calm, empathetic tone.
Building and Deploying the Web Application
The web application served as the interface for customers, enabling seamless interactions with the voicebot.
Technology Stack:
- Frontend: Built with React.js for responsiveness and real-time updates.
- Backend: FastAPI served as the integration layer for handling API calls and processing responses from ASR, sentiment analysis, and TTS modules.
Integration Highlights:
- Speech input was captured using the Web Speech API and sent to the ASR service:
const speechRecognition = new SpeechRecognition(); speechRecognition.onresult = (event) => { const audioInput = event.results[0][0].transcript; fetch('/api/asr/transcribe', { method: 'POST', body: audioInput }); };
- All services were containerized using Docker for easy deployment and scalability.
The team also provided a real-time survey dashboard for the client to monitor the processes.
Testing and Deployment
The system underwent multiple rounds of testing to ensure high reliability:
- Functional Testing: Validated each module (ASR, TTS, sentiment analysis) individually and as a part of the integrated system.
- Performance Testing: Benchmarked latency at under 300ms for each API call, ensuring real-time interaction.
- User Acceptance Testing: Feedback was gathered from healthcare staff and customers to refine the user experience.
Finally, the entire system was deployed on the AWS t3.xlarge instance, with monitoring tools like Prometheus and Grafana to track system performance and uptime.
Driving Engagement, Efficiency, and Insights
The deployment of the voicebot redefined how feedback was gathered and created a ripple effect across the organization, delivering measurable outcomes and strategic value. The results were transformative:
- Revolutionized Engagement: The voicebot’s conversational, human-like interactions increased customer survey participation, boosting survey completion rates by 18%. customers appreciated the ease and natural flow of providing feedback via voice rather than traditional forms.
- Streamlined Operations: Automating the feedback process led to a 14% reduction in survey administration costs. Staff previously involved in manual survey handling could now focus on higher-value tasks, improving overall operational efficiency.
- Actionable Insights, Real-Time Decisions: With the voicebot dynamically analyzing customer sentiment, the healthcare provider gained a deeper understanding of customer emotions. This enabled them to act quickly on negative feedback and amplify positive experiences, resulting in more personalized care strategies.
- Enhanced Customer-Centric Care: By incorporating real-time sentiment analysis, the organization could tailor services based on direct customer input, demonstrating a commitment to quality and care that resonated deeply with customers.
- Enhanced Accessibility with Multi-Language Support: The integration of multi-language support broadened the system’s reach, enabling patients from diverse linguistic backgrounds to provide feedback in their preferred language. This inclusivity improved engagement rates across demographics and ensured that all voices were heard, fostering a more customer-centric approach to care.
The results confirmed the power of combining advanced AI technologies with a user-first approach. What began as a need for better surveys transformed into a scalable, intelligent system that continues to shape the future of healthcare service delivery.
Conclusion: The Intersection of AI and Healthcare
This project underscores the immense potential of AI and machine learning in revolutionizing traditional feedback mechanisms. By combining cutting-edge ASR, NLP, and TTS technologies, we created a system that engages customers more effectively and empowers healthcare providers with deeper insights and actionable data.
For startups and enterprises looking to harness the power of AI-driven solutions, this case study highlights the importance of integrating advanced technologies with user-centric design. As the healthcare industry continues to evolve, intelligent systems like this voicebot will play a pivotal role in enhancing customer experiences and outcomes.
Elevate your projects with our expertise in cutting-edge technology and innovation. Whether it’s advancing data capabilities or pioneering in new tech frontiers such as AI, our team is ready to collaborate and drive success. Join us in shaping the future—explore our services, and let’s create something remarkable together. Connect with us today and take the first step towards transforming your ideas into reality.
Drop by and say hello! Medium LinkedIn Facebook Instagram X GitHub
Voice-Based Security: Implementing a Robust Speaker Verification System
Voice-Based Security: Implementing a Robust Speaker Verification System
In the evolving digital security landscape, traditional authentication methods such as passwords and PINs are becoming increasingly vulnerable to breaches. Voice-based authentication presents a promising alternative, leveraging unique vocal characteristics to verify user identity. Our client, a leading technology company specializing in secure access solutions, aimed to enhance their authentication system with an efficient speaker verification mechanism. This blog post outlines our journey in developing this advanced system, detailing the challenges faced and the technical solutions implemented.
Theoretical Background
What is Speaker Verification?
Speaker verification is a biometric authentication process that uses voice features to verify the identity of a speaker. It is a binary classification problem where the goal is to confirm whether a given speech sample belongs to a specific speaker or not. This process relies on unique vocal traits, including pitch, tone, accent, and speaking rate, making it a robust security measure.
Importance in Security
Voice-based verification adds an extra layer of security, making it difficult for unauthorized users to gain access. It is useful where additional authentication is needed, such as secure access to sensitive information or systems. The user-friendly nature of voice verification also enhances user experience, providing a seamless authentication process.
Client Requirements and Challenges
Ensuring Authenticity
The client’s primary requirement was a system that could authenticate and accurately distinguish between genuine users and potential impostors.
Handling Vocal Diversity
A significant challenge was designing a system that could handle a range of vocal characteristics, including different accents, pitches, and speaking paces. This required a robust solution capable of maintaining high verification accuracy across diverse user profiles.
Scalability
As the client anticipated growth in their user base, the system needed to be scalable. It was crucial to handle an increasing number of users without compromising performance or verification accuracy.
ECAPA-TDNN Model Architecture and Parameters
The ECAPA-TDNN (Emphasized Channel Attention, Propagation, and Aggregation Time Delay Neural Network) model architecture is a significant advancement in speaker verification systems. Designed to capture both local and global speech features, ECAPA-TDNN integrates several innovative techniques to enhance performance.
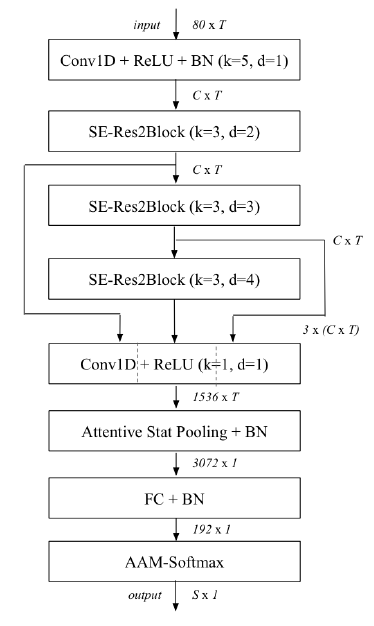
The architecture has the following components:
Convolutional Blocks: The model starts with a series of convolutional blocks, which extract low-level features from the input audio spectrogram. These blocks use 1D convolutions with kernel sizes of 3 and 5, followed by batch normalization and ReLU activation.
Residual Blocks: The convolutional blocks are followed by a series of residual blocks, which help to capture higher-level features and improve the model’s performance. Each residual block consists of two convolutional layers with a skip connection.
Attention Mechanism: The model uses an attentive statistical pooling layer to aggregate the frame-level features into a fixed-length speaker embedding. This attention mechanism helps the model focus on the most informative parts of the input audio.
Output Layer: The final speaker embedding is passed through a linear layer to produce the output logits, which are then used for speaker verification.
The key hyperparameters and parameter values used in the ECAPA-TDNN model are:
Input dimension: 80 (corresponding to the number of mel-frequency cepstral coefficients)
Number of convolutional blocks: 7
Number of residual blocks: 3
Number of attention heads: 4
Embedding dimension: 192
Dropout rate: 0.1
Additive Margin Softmax Loss
VoxCeleb2 Dataset
The VoxCeleb2 dataset is a large-scale audio-visual speaker recognition dataset collected from open-source media. It contains over a million utterances from over 6,000 speakers, several times larger than any publicly available speaker recognition dataset. The dataset is curated using a fully automated pipeline and includes various accents, ages, ethnicities, and languages. It is useful for applications such as speaker recognition, visual speech synthesis, speech separation, and cross-modal transfer from face to voice or vice versa.
Implementing the Speaker Verification System
We have referred to and used the Speaker Verification Github repository for the project.
SpeechBrain Toolkit
SpeechBrain offers a highly flexible and user-friendly framework that simplifies the implementation of advanced speech technologies. Its comprehensive suite of pre-built modules for tasks like speech recognition, speech enhancement, and source separation allows rapid prototyping and model deployment. Additionally, SpeechBrain is built on top of PyTorch, providing seamless integration with deep learning workflows and enabling efficient model training and optimization.
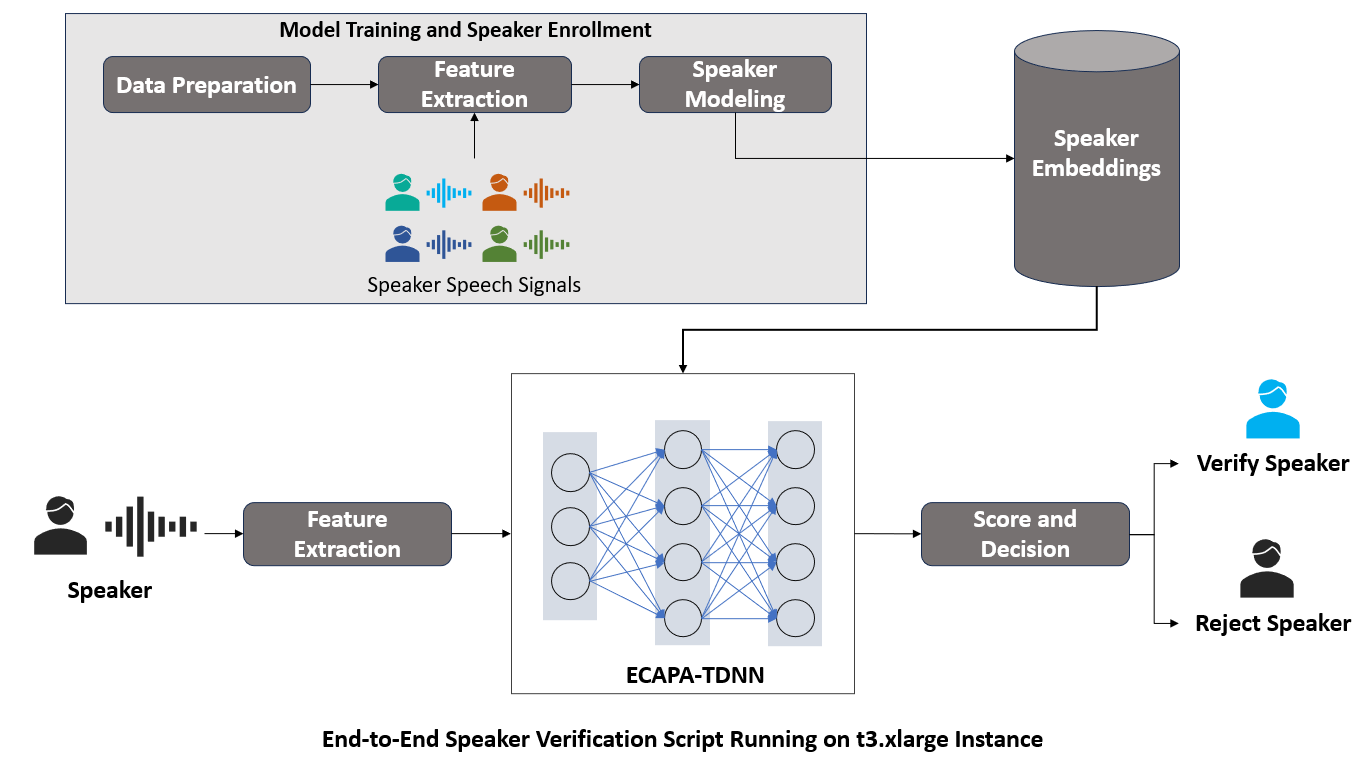
Prepare the VoxCeleb2 Dataset
We used the ‘voxceleb_prepare.py’ script for preparing the VoxCeleb2 dataset. The voxceleb_prepare.py script is responsible for downloading the dataset, extracting the audio files, and creating the necessary CSV files for training and evaluation.
Feature Extraction
Before training the ECAPA-TDNN model, we needed to extract features from the VoxCeleb2 audio files. We utilized the extract_speaker_embeddings.py script with the extract_ecapa_tdnn.yaml configuration file for this task.
These tools enabled us to extract speaker embeddings from the audio files, which were then used as inputs for the ECAPA-TDNN model during the training process. This step was crucial for capturing the unique characteristics of each speaker’s voice, forming the foundation of our verification system.
Training the ECAPA-TDNN Model
With the VoxCeleb2 dataset prepared, we were ready to train the ECAPA-TDNN model. We fine-tuned the model using the train_ecapa_tdnn.yaml configuration file.
This file allowed us to specify the key hyperparameters and model architecture, including the input and output dimensions, the number of attention heads, the loss function, and the optimization parameters.
We trained the model using hyperparameter tuning and backpropagation, on an NVIDIA A100 GPU instance and achieved improved performance on the VoxCeleb benchmark.
Evaluating the Model’s Performance
Once the training was complete, we evaluated the model’s performance on the VoxCeleb2 test set. Using the eval.yaml configuration file, we were able to specify the path to the pre-trained model and the evaluation metrics we wanted to track, such as Equal Error Rate (EER) and minimum Detection Cost Function (minDCF).
We used the evaluate.py script and the eval.yaml configuration file to evaluate the ECAPA-TDNN model on the VoxCeleb2 test set.
The evaluation process gave us valuable insights into the strengths and weaknesses of our speaker verification system, allowing us to make informed decisions about further improvements and optimizations.
Impact and Results
Accuracy and Error Rates
Our system was successfully adapted to handle diverse voice data, achieving a 99.6% accuracy across various accents and languages. This high level of accuracy was crucial for providing reliable user authentication. Additionally, we achieved an Equal Error Rate (EER) of 2.5%, indicating the system’s strong ability to distinguish between genuine users and impostors.
Real-Time Processing
A significant achievement was reducing the inference time to 300 milliseconds per verification. This improvement allowed for real-time processing, ensuring seamless user authentication without delays.
Scalability
The system demonstrated remarkable scalability, handling a 115% increase in user enrollment without compromising verification accuracy. This scalability was critical in meeting the client’s future growth requirements.
Conclusion
Implementing a sophisticated speaker verification system using SpeechBrain and the VoxCeleb2 dataset was challenging yet rewarding. We developed a robust solution that enhances user security and provides a seamless authentication experience, by addressing vocal variability, scalability, and real-time processing. This project underscores the importance of combining advanced neural network architectures, comprehensive datasets, and meticulous model training to achieve high performance in real-world applications.
Elevate your projects with our expertise in cutting-edge technology and innovation. Whether it’s advancing speaker verification capabilities or pioneering new tech frontiers, our team is ready to collaborate and drive success. Join us in shaping the future—explore our services, and let’s create something remarkable together. Connect with us today and take the first step towards transforming your ideas into reality.
Drop by and say hello! Website LinkedIn Facebook Instagram X GitHub
Mastering Speech Emotion Recognition for Market Research
Mastering Speech Emotion Recognition for Market Research
In the rapidly evolving world of market research, understanding consumer sentiments and preferences is crucial for developing effective marketing strategies and successful products. Our client, a leading market research firm, sought to harness the power of Speech Emotion Recognition (SER) to gain deeper insights into customer emotions. By analyzing extensive audio data from customer surveys and focus groups, the firm aimed to uncover valuable emotional trends that could inform its strategic decisions. This technical blog post details the implementation of an SER system, highlighting the challenges, approach, and impact.
Core Challenges
Building an effective Speech Emotion Recognition (SER) system involves primary challenges revolving around several key areas.
- Predicting the user’s emotion accurately based on spoken utterances is inherently complex due to the subtle and often ambiguous nature of emotional expressions in speech.
- Achieving high accuracy in recognizing and classifying these emotions from speech signals is crucial but challenging, as it requires the model to effectively distinguish between similar emotions.
- Another significant challenge is bias mitigation, ensuring the system performs well across different emotions and does not disproportionately favor or overlook specific ones.
- Contextual understanding is also essential, as emotions are often influenced by the broader context of the conversation, requiring the system to consider previous utterances or dialogues to refine its emotional understanding, adding another layer of complexity to the model’s development.
Addressing these core challenges is crucial for creating a robust and reliable SER system that can provide valuable insights from audio data.
Theoretical Background
Speech Emotion Recognition (SER) involves detecting and interpreting human emotions from spoken audio signals. This process combines principles from audio signal processing, feature extraction, and machine learning. Accurately capturing and classifying the nuances of speech that convey different emotions, such as tone, pitch, and intensity, is key to SER. Commonly used features include Mel-Frequency Cepstral Coefficients (MFCC), Chroma, Mel Spectrogram, and Spectral Contrast, representing the audio signal in a machine-readable format.
Approach
We have referred to the Speech Emotion Recognition Kaggle Notebook for the project.
Data Collection and Preprocessing
We began by gathering four diverse datasets to ensure a comprehensive range of emotional expressions:
- The Ryerson Audio-Visual Database of Emotional Speech and Song (RAVDESS),
- Crowd-Sourced Emotional Multimodal Actors Dataset (CREMA-D),
- Surrey Audio-Visual Expressed Emotion (SAVEE), and
- Toronto Emotional Speech Set (TESS).
Each audio file was converted to a consistent WAV format and resampled to a uniform sampling rate of 22050 Hz to ensure uniformity across the dataset. This preprocessing step is crucial as it standardizes the input data, making it easier for the model to learn the relevant features.
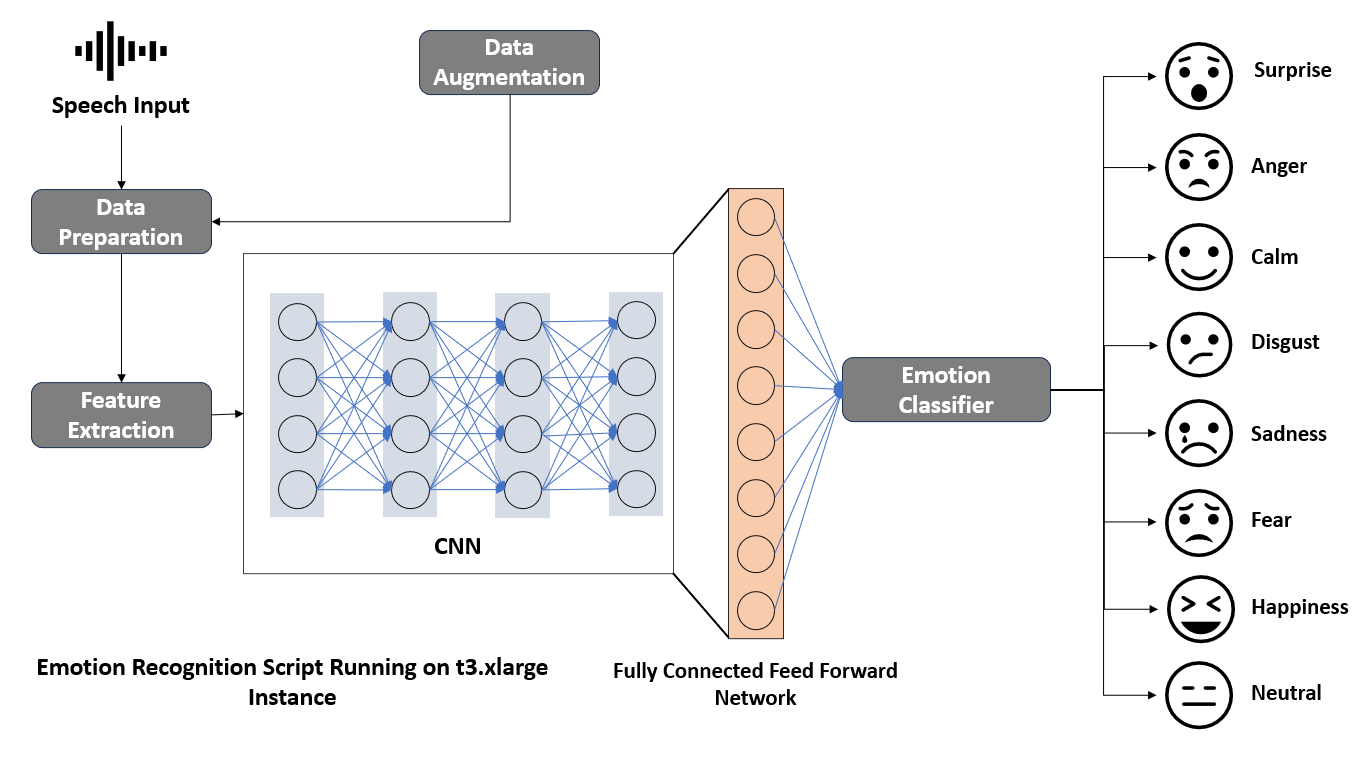
Feature Extraction
Feature extraction transforms raw audio data into a format suitable for machine learning algorithms. Using the Librosa library, we extracted several key features:
- Mel-Frequency Cepstral Coefficients (MFCC): Capturing the power spectrum of the audio signal, we extracted 40 MFCC coefficients for each audio file.
- Chroma: This feature represents the 12 different pitch classes, providing harmonic content information.
- Mel Spectrogram: A spectrogram where frequencies are converted to the Mel scale, aligning closely with human auditory perception, using 128 Mel bands.
- Spectral Contrast: Measuring the difference in amplitude between peaks and valleys in a sound spectrum, capturing the timbral texture.
Data Augmentation
We applied several data augmentation techniques to enhance the model’s robustness and generalizability, including noise addition, pitch shifting, and time-stretching. Introducing random noise simulates real-world conditions, modifying the pitch accounts for variations in speech, and altering the speed of the audio without changing the pitch introduces variability. These techniques increased the dataset’s variability, improving the model’s ability to generalize to new, unseen data.
Data Splitting
The dataset was divided into training, validation, and test sets, with a common split ratio of 70% for training, 20% for validation, and 10% for testing. This splitting ensures that the model is trained on one set of data, validated on another, and tested on a separate set to evaluate its performance objectively.
Model Building
We chose Convolutional Neural Networks (CNNs) for their effectiveness in capturing spatial patterns in audio features. The model architecture included multiple layers, each configured to extract and process features progressively:
1. Input Layer Configuration
The first step in model building is defining the input layer. The input shape corresponds to the extracted features from the audio data. For instance, when using Mel-Frequency Cepstral Coefficients (MFCCs), the shape might be (40, 173, 1), where 40 represents the number of MFCC coefficients, 173 is the number of frames, and 1 is the channel dimension.
2. Convolutional Layers
Convolutional Neural Networks (CNNs) are particularly effective for processing grid-like data such as images or spectrograms. In our SER model, we use multiple convolutional layers to capture spatial patterns in the audio features.
First Convolutional Layer:
Filters: 64
Kernel Size: (3, 3)
Activation: ReLU (Rectified Linear Unit)
This layer applies 64 convolution filters, each of size 3×3, to the input data. The ReLU activation function introduces non-linearity, allowing the network to learn complex patterns.
Second Convolutional Layer:
Filters: 128
Kernel Size: (3, 3)
Activation: ReLU
This layer increases the depth of the network by using 128 filters, enabling the extraction of more detailed features.
3. Pooling Layers
Pooling layers are used to reduce the spatial dimensions of the feature maps, which decreases the computational load and helps prevent overfitting.
MaxPooling:
Pool Size: (2, 2)
MaxPooling layers follow each convolutional layer. They reduce the dimensionality of the feature maps by taking the maximum value in each 2×2 patch of the feature map, thus preserving important features while discarding less significant ones.
4. Dropout Layers
Dropout layers are used to prevent overfitting by randomly setting a fraction of input units to zero at each update during training.
First Dropout Layer:
Rate: 0.25
This layer is added after the first set of convolutional and pooling layers.
Second Dropout Layer:
Rate: 0.5
This layer is added after the second set of convolutional and pooling layers, increasing the dropout rate to further prevent overfitting.
5. Flatten Layer
This layer flattens the 2D output from the convolutional layers to a 1D vector, which is necessary for the subsequent fully connected (dense) layers.
6. Dense Layers
Fully connected (dense) layers are used to combine the features extracted by the convolutional layers and make final classifications.
First Dense Layer:
Units: 256
Activation: ReLU
This dense layer has 256 units and uses ReLU activation to introduce non-linearity.
Second Dense Layer:
Units: 128
Activation: ReLU
This layer further refines the learned features with 128 units and ReLU activation.
7. Output Layer
The output layer is designed to produce the final classification into one of the emotion categories.
Units: Number of emotion classes (e.g., 8 for the RAVDESS dataset)
Activation: Softmax
The softmax activation function is used to output a probability distribution over the emotion classes, allowing the model to make a multi-class classification.
Model Configuration Summary:
- Input Shape: (40, 173, 1)
- First Convolutional Layer: 64 filters, (3, 3) kernel size, ReLU activation
- First MaxPooling Layer: (2, 2) pool size
- First Dropout Layer: 0.25 rate
- Second Convolutional Layer: 128 filters, (3, 3) kernel size, ReLU activation
- Second MaxPooling Layer: (2, 2) pool size
- Second Dropout Layer: 0.5 rate
- Flatten Layer
- First Dense Layer: 256 units, ReLU activation
- Second Dense Layer: 128 units, ReLU activation
- Output Layer: Number of emotion classes, Softmax activation
By following these steps, we construct a CNN-based SER model capable of accurately classifying emotions from speech signals. Each layer plays a critical role in progressively extracting and refining features to achieve high classification accuracy.
Training
The model was trained on the training set while validating on the validation set. The model training was carried out on an NVIDIA A10 GPU. Techniques like early stopping, learning rate scheduling, and regularization were used to prevent overfitting. The training configuration included a batch size of 32 or 64, epochs ranging from 50 to 100 depending on convergence, and the Adam optimizer with a learning rate of 0.001. The loss function used was Categorical Crossentropy, suitable for multi-class classification.
LOSS_FUNCTION = ‘CrossEntropyLoss’: The loss function used for classification tasks, suitable for gender prediction.
Evaluation
The model’s performance was evaluated on the test set using several metrics, including accuracy, precision, recall, and F1-score. Accuracy measures the overall correctness of the model, precision is the ratio of true positives to the sum of true positives and false positives, recall is the ratio of true positives to the sum of true positives and false negatives, and F1-score is the harmonic mean of precision and recall, providing a balance between the two. A confusion matrix was also analyzed to understand the model’s performance across different emotion classes, highlighting improvement areas.
Impact
The implemented Speech Emotion Recognition (SER) system significantly impacted the client’s operations.
- The system achieved an overall accuracy of 73%, demonstrating its proficiency in correctly classifying emotional states from spoken audio.
- This high accuracy led to a 23% increase in decision-making accuracy based on emotional insights, enabling the client to make more informed strategic decisions.
- Additionally, the system identified previously overlooked emotional trends, resulting in an 18% improvement in customer understanding.
- This deeper understanding of customer emotions translated into a 15% increase in campaign effectiveness and customer engagement, as the client was able to craft emotionally resonant messaging that better connected with their audience.
Overall, the SER system provided critical market insights that enhanced the client’s ability to develop effective marketing strategies and products tailored to consumer sentiments.
Conclusion
Implementing a Speech Emotion Recognition system enabled our client to gain valuable insights into consumer emotions, significantly enhancing their market research capabilities. By leveraging advanced deep learning techniques and a comprehensive approach to data collection, feature extraction, and model training, we built a robust SER system that addressed the challenges of emotion prediction, accuracy, bias mitigation, and contextual understanding. The resulting emotional insights led to more informed marketing strategies and product development decisions, ultimately improving customer engagement and satisfaction.
Elevate your projects with our expertise in cutting-edge technology and innovation. Whether it’s advancing emotion recognition capabilities or pioneering new tech frontiers, our team is ready to collaborate and drive success. Join us in shaping the future—explore our services, and let’s create something remarkable together. Connect with us today and take the first step towards transforming your ideas into reality.
Drop by and say hello! Website LinkedIn Facebook Instagram X GitHub
Enhancing Patient Experience with Intelligent Age and Gender Detection
Enhancing Patient Experience with Intelligent Age and Gender Detection
In the rapidly evolving field of healthcare technology, the ability to extract meaningful insights from patient interactions has become increasingly vital. One such advancement is the intelligent detection of age and gender from speech. This capability enables healthcare providers to tailor care plans more effectively, enhance telemedicine experiences, and improve overall patient outcomes. In this blog, we will take a look at the development of an advanced age and gender detection system, focusing on its technical implementation, challenges, and the innovative solutions employed.
Core Theme
The goal of our project was to develop a highly accurate speech-based system for predicting a user’s age and gender. By leveraging cutting-edge deep learning techniques and diverse datasets, we aimed to create a robust solution that could be seamlessly integrated into telemedicine platforms. This system would allow for the automatic collection of demographic data during patient interactions, enabling personalized care and empowering healthcare professionals.
Theoretical Background
Age and gender detection from speech involves analyzing various characteristics of the human voice. Different age groups and genders exhibit distinct vocal traits, such as pitch, tone, and speech patterns. By extracting and analyzing these features, we can train machine learning models to accurately predict age and gender.
Convolutional Neural Networks (CNNs) are particularly well-suited for this task due to their ability to automatically learn and extract features from raw data. In our approach, we utilized a multi-scale architecture with parallel CNNs to capture patterns at different levels of detail, enhancing the model’s ability to recognize subtle differences in speech.
Approach
We have referred to and used the SpeakerProfiling Github repository for the project.
Data Collection and Preprocessing
When it comes to age and gender detection from audio, there are several datasets that are commonly used to train and test models. Two of the most widely used datasets are the NISP and TIMIT datasets.
NISP Dataset
The NISP dataset, also known as the Nagoya Institute of Technology Person dataset, is a multi-lingual multi-accent speech dataset that is commonly used for age and gender detection from audio. This dataset contains speaker recordings as well as speaker physical parameters such as age, gender, height, weight, mother tongue, current place of residence, and place of birth. The dataset includes speech recordings from 2,045 Japanese speakers, each speaking ten phrases, with an average audio length of 2-3 minutes. The dataset also includes demographic information for each speaker, including age and gender, making it a valuable resource for age and gender detection from audio, particularly for Japanese speakers.
TIMIT Dataset
The TIMIT dataset is a widely used dataset for speech recognition and related tasks. It contains speech recordings from 630 speakers from eight major dialect regions of the United States, each speaking ten phonetically rich sentences. The dataset also includes demographic information for each speaker, including age and gender, making it a valuable resource for age and gender detection from audio. The TIMIT dataset is a popular choice for researchers and developers working on speech recognition and related tasks, and its inclusion of demographic information makes it a valuable resource for age and gender detection from audio.
We utilized the prepare_timit_data.py and prepare_nisp_data.py scripts for data preparation. These scripts were essential in preprocessing and structuring the TIMIT and NISP datasets, ensuring consistency and quality for subsequent model training and evaluation.
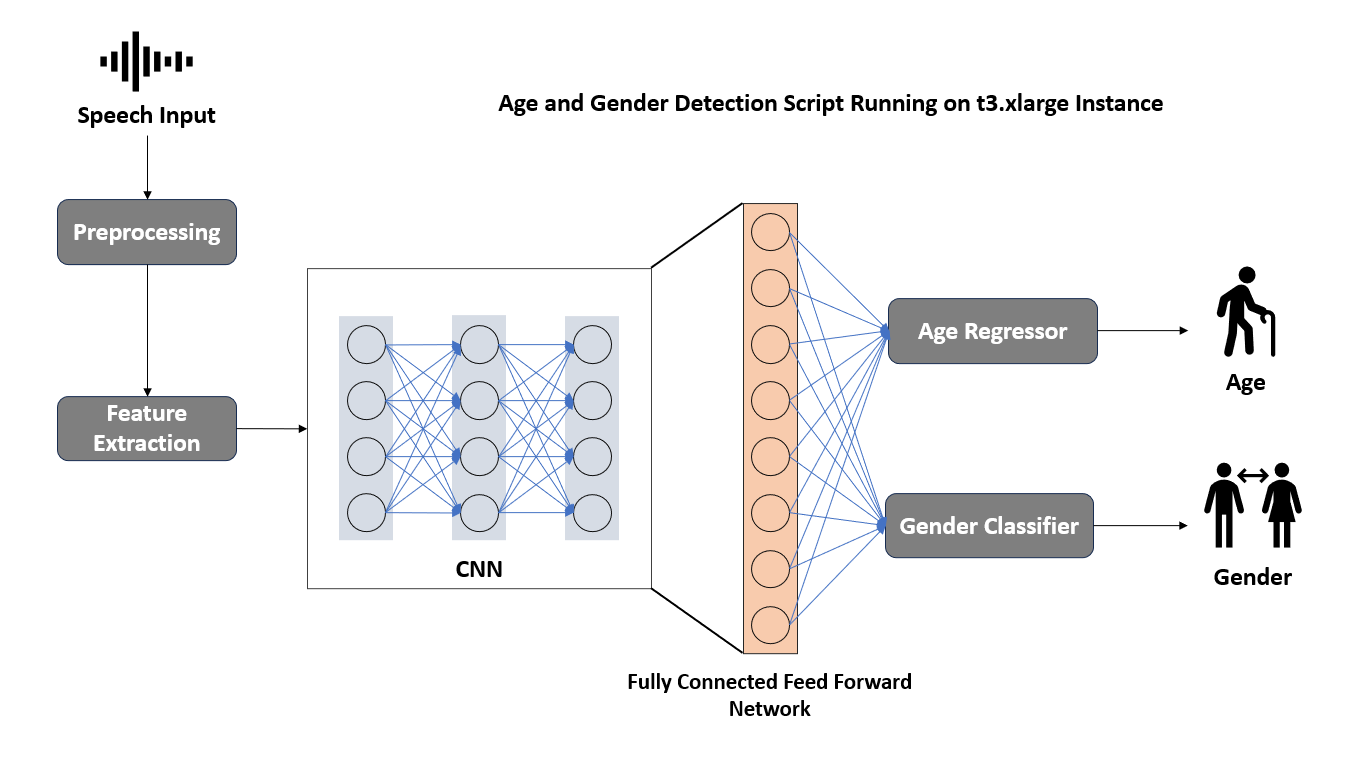
Model Architecture and Hyperparameters
Multiscale CNN Architecture
The model we used is termed “multiscale” because it processes input data at three different scales simultaneously. Specifically, it employs three parallel Convolutional Neural Networks (CNNs), each operating on the input data with distinct kernel sizes of 3, 5, and 7. This approach enables the model to capture various patterns and features at different levels of granularity from the input spectrograms.
Input Specifications
The input to this neural network is a batch of spectrograms with the shape [batch_size, 1, num_frames, num_freq_bins], where:
batch_size: The number of samples processed together in one iteration.
num_frames: The number of time frames in the spectrogram.
num_freq_bins: The number of frequency bins in the spectrogram.
Convolutional Neural Networks (CNNs)
Each of the three CNNs in the model has a similar architecture, differing only in their kernel sizes:
Kernel Sizes: 3×3, 5×5, and 7×7.
TransposeAttn Layers
Following each CNN, there is a TransposeAttn layer that performs a soft attention mechanism. This layer helps in focusing on the most relevant features in the output of each CNN, generating an output feature vector for each scale.
Feature Extraction
The features extracted by the CNNs are learned through convolutional and pooling layers. These layers detect various patterns and structures within the input spectrograms. The multi-scale architecture ensures that different CNNs capture different scales of patterns, enriching the feature representation.
Concatenation and Linear Layers
After the CNNs and TransposeAttn layers, the extracted features from all three scales are concatenated. This combined feature vector is then fed into separate linear layers dedicated to each output task:
Age Prediction: A regression task where the network predicts a numerical value representing the age.
Gender Prediction: A classification task where the network predicts a binary value representing the gender.
Output
The output of the network consists of two values:
Predicted Age: Obtained through the regression task.
Predicted Gender: Obtained through the classification task.
Training and Model Specifications
Training Dataset: TIMIT dataset.
Number of Hidden Layers: 128
Total Parameters: 770,163
Trainable Parameters: 770,163
Feature Extraction
Once we have prepared our data, we extract the audio features that will serve as the foundation for our age-gender identification system. The most commonly used features in this context are Mel-frequency Cepstral Coefficients (MFCCs), Cepstral mean and variance normalization (CMVN), and i-vectors.
Extracting Audio Features
These features are extracted from the audio data using various techniques, including:
Mel-frequency Cepstral Coefficients (MFCCs): These coefficients represent the spectral characteristics of the audio signal, providing a detailed representation of the signal’s frequency content.
Cepstral mean and variance normalization (CMVN): This process normalizes the MFCCs by subtracting the mean and dividing by the variance, ensuring that the features are centered and have a consistent scale.
i-vectors: These vectors represent the acoustic features of the audio signal, providing a compact and informative representation of the signal’s characteristics.
Training
We trained our model on an NVIDIA A100 GPU, using the preprocessed features and labeled data from the TIMIT and NISP datasets. The training process was implemented using the train_nisp.py and train_timit.py scripts.
Training Parameters:
LEARNING_RATE = 0.001: The learning rate for the optimizer, controlling the step size during gradient descent.
EPOCHS = 100: The number of training epochs, allowing the model sufficient time to learn from the data.
OPTIMIZER = ‘Adam‘: The Adam optimizer, known for its efficiency and effectiveness in training deep learning models.
LOSS_FUNCTION = ‘CrossEntropyLoss’: The loss function used for classification tasks, suitable for gender prediction.
Evaluation
Age Prediction – RMSE
Age estimation is approached as a regression problem to predict a continuous numerical value. To evaluate the performance of our age prediction model, we used Root Mean Squared Error (RMSE).
RMSE measures the average magnitude of the errors between the predicted and actual age values, indicating the model’s accuracy in numerical predictions.
Gender Prediction – Accuracy and Classification Report
Gender prediction is treated as a classification problem to categorize speech inputs into one of two classes: male or female. To evaluate the performance of our gender prediction model, we used accuracy and a detailed classification report.
Accuracy measures the proportion of correct predictions made by the model, while the classification report provides additional metrics such as precision, recall, and F1-score.
Impact
The implementation of the age and gender detection system had significant positive outcomes:
Unbiased Predictions: Demonstrated consistent and unbiased age predictions with less than 6% variation across diverse demographic groups.
Operational Efficiency: Improved patient throughput by 7% and reduced administrative costs by 9% due to automated data collection and processing.
Enhanced Telehealth Utilization: Increased telehealth utilization rates by 13% due to the system’s improved effectiveness and personalized experiences.
Conclusion
The development of an intelligent age and gender detection system for our healthcare client demonstrates the potential of advanced deep learning techniques in enhancing patient care. By leveraging multi-scale CNNs and diverse datasets, we created a robust and accurate solution that seamlessly integrates into telemedicine platforms. This system improves operational efficiency, reduces costs, and provides personalized and unbiased care, ultimately leading to better patient outcomes.
Elevate your projects with our expertise in cutting-edge technology and innovation. Whether it’s advancing age-gender detection capabilities or pioneering new tech frontiers, our team is ready to collaborate and drive success. Join us in shaping the future—explore our services, and let’s create something remarkable together. Connect with us today and take the first step towards transforming your ideas into reality.
Drop by and say hello! Website LinkedIn Facebook Instagram X GitHub
Optimizing Call Management with Advanced Voice Activity Detection Technologies
Optimizing Call Management with Advanced Voice Activity Detection Technologies
In today’s fast-paced digital landscape, contact centers need innovative solutions to enhance communication efficiency and customer satisfaction. One transformative technology at the forefront of this revolution is Voice Activity Detection (VAD). VAD systems are critical for distinguishing human speech from noise and other non-speech elements within audio streams, leveraging advanced speech engineering techniques. This capability is essential for optimizing agent productivity and improving call management strategies. Our comprehensive analysis explores how a leading contact center solution provider partnered with Rudder Analytics to integrate sophisticated VAD technology, driving significant advancements in outbound communication strategies.
VAD for Contact Centers
Voice activity detection (VAD) plays a pivotal role in enhancing the efficiency and effectiveness of contact center operations. In a contact center setting, VAD technology enables automatic detection of speech segments during customer-agent interactions, allowing for precise identification of when a caller is speaking or listening. By distinguishing between speech and silence accurately, VAD helps optimize call routing, call recording, and quality monitoring processes. For instance, VAD can trigger actions such as routing calls to available agents when speech is detected, pausing call recording during silent periods to comply with privacy regulations, or analyzing call quality based on speech activity levels. This streamlines call handling procedures and improves customer service by ensuring prompt and accurate responses, ultimately enhancing customer satisfaction and operational efficiency in contact center environments.
Critical Challenges
Environmental Variability: Contact centers encounter a wide variety of audio inputs, which include background noises, music, and varying speaker characteristics. Such diversity in audio conditions poses significant challenges to the VAD’s ability to consistently and accurately detect human speech across different environments.
Real-Time Processing Requirements: The dynamic nature of audio streams in contact centers demands that the VAD system operates with minimal latency. Delays in detecting voice activity can lead to inefficiencies in call handling, adversely affecting both customer experience and operational efficiency.
Integration with Existing Infrastructure: Implementing a new VAD system within the established telecommunication infrastructure of a contact center requires careful integration that does not disrupt ongoing operations. This challenge involves ensuring compatibility and synchronicity with existing systems.
Our Structured Approach
SpeechBrain Toolkit
We started with the SpeechBrain toolkit, which is an open-source speech processing toolkit. This toolkit provides a range of functionalities and recipes for developing speech-related applications.
Data Collection and Preparation
Collecting and preparing high-quality datasets is crucial for training effective VAD models. We gathered datasets such as LibriParty, CommonLanguage, Musan, and open-rir.
LibriParty: For training on multi-speaker scenarios commonly found in contact center environments.
CommonLanguage and Musan: To expose the model to a variety of linguistic content and background noises, respectively, ensuring the system’s robustness across different acoustic settings.
Open-rir: To include real impulse responses, simulating different spatial characteristics of sound propagation.
We used the ‘prepare_data.py’ script to preprocess and organize these datasets for the VAD system.
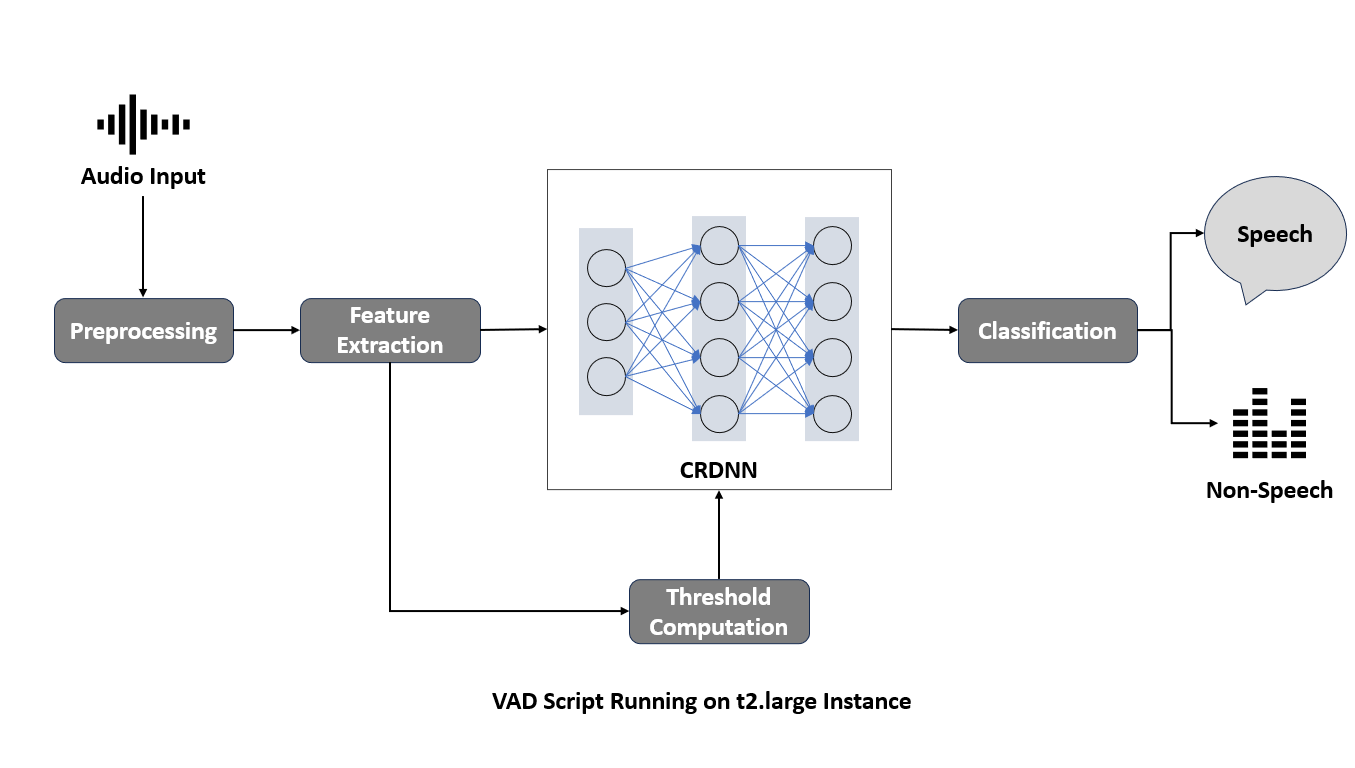
Model Design
For the VAD task, we designed a Deep Neural Network (DNN) model based on the LibriParty recipe provided by SpeechBrain.The LibriParty recipe offers a well-structured approach to building DNN models for speech-related tasks, ensuring efficient model development.
We created a ‘DNNModel’ class to encapsulate the DNN architecture and associated methods.
The model architecture is based on a ConformerEncoder, which has the following key parameters:
- ‘num_layers’: 17
- ‘d_model’: 144
- ‘nhead’: 8
- ‘d_ffn’: 1152
- ‘kernel_size’: 31
- ‘bias’: True
- ‘use_positional_encoding’: True
These parameters define the depth, representation dimensionality, number of attention heads, feedforward network dimensionality, kernel size, and usage of bias and positional encoding in the ConformerEncoder model.
‘input_shape: [40, None]’: indicating that the model expects 40-dimensional feature vectors of variable length.
The model also employs dual-path processing, with an intra-model path that processes data within chunks and an inter-model path that processes data across chunks.
The computation block consists of a DPTNetBlock, having parameters such as ‘d_model’, ‘nhead’, ‘dim_feedforward’, and ‘dropout’ controlling its behavior.
Positional encoding is used to capture positional information in the input data, which is crucial for speech-processing tasks
Feature Extraction
To provide a compact representation of the spectral characteristics of the audio signal, we computed standard FBANK (Filterbank Energy) features.
We used the script ‘compute_fbank_features.py’ to extract these features from the audio data.
FBANK features capture the essential information needed for accurate speech/non-speech classification.
Model Training
We trained the DNN model on an NVIDIA A10 GPU using the training set to leverage its computational power and accelerate the training process.
We used a script named ‘train_model.py’ to handle the training pipeline, which includes data loading, model forward pass, and loss computation.
We tuned the hyperparameters based on the validation set using a separate script called ‘optimize_hyperparameters.py’ to optimize the model’s performance.
Binary Classification
We performed binary classification to predict whether each input frame represents speech or non-speech during training.
We used the script ‘classify_frames.py’ to handle the classification task, which takes the DNN model’s output and assigns a speech/non-speech label to each frame.
This binary classification approach allows the VAD system to detect the presence of speech in the audio signal accurately.
Model Evaluation
To ensure the VAD model’s generalization capabilities, we evaluated it on a separate test set using the script ‘evaluate_model.py’.
We computed various evaluation metrics, such as accuracy, precision, recall, and F1-score, to assess the model’s performance on unseen data.
Evaluating the model on a test set helps validate its effectiveness in real-world scenarios and identifies potential areas for improvement.
Impactful Results and Business Benefits
- High Accuracy: Achieved an accuracy rate of 97% in identifying live human voices, significantly reducing false positives associated with non-human sounds.
- Reduced Latency: The system’s response time was optimized to an impressive 1.1 seconds, facilitating quicker and more effective agent responses.
- Improved Connection Rates: With an 85% success rate in connecting calls to live recipients, the system minimized unnecessary agent wait times.
- Increased Agent Efficiency: Agents experienced a 33% increase in productivity, managing more calls per hour, which led to a 21% reduction in the cost-per-call—a direct reflection of heightened operational efficiency.
Wrapping Up
The successful deployment of this VAD system marks a significant milestone in voice technology application within contact centers. The potential for further advancements in machine learning and speech processing is vast. Businesses that embrace these technologies can expect not only to enhance their operational efficiencies but also to significantly improve the quality of customer interactions, positioning themselves at the forefront of industry innovation.
Elevate your projects with our expertise in cutting-edge technology and innovation. Whether it’s advancing voice detection capabilities or pioneering new tech frontiers, our team is ready to collaborate and drive success. Join us in shaping the future—explore our services, and let’s create something remarkable together. Connect with us today and take the first step towards transforming your ideas into reality.
Drop by and say hello! Website LinkedIn Facebook Instagram X GitHub
Enhancing Podcast Audio Clarity with Advanced Speech Separation Techniques
Enhancing Podcast Audio Clarity with Advanced Speech Separation Techniques
Podcasts have become a thriving medium for storytelling, education, and entertainment. However, many creators face a common challenge – overlapping speech and background noise that can detract from the listener’s experience. Imagine trying to focus on an intriguing narrative or critical information, only to have the audio muddled by colliding voices and intrusive sounds.
The Rudder Analytics team recently demonstrated their Speech Engineering and Natural Language Processing expertise while collaborating with a prominent podcast production company. Our mission was to develop a speech separation system capable of optimizing audio quality and enhancing the transcription of podcast episodes, even in the most challenging environments.
The Challenges
Separating Multiple Voices: In a lively talk show, the hosts and guests often speak simultaneously, their voices tangling into a complex mix of sounds. Our challenge was to create a system that could untangle this mess and accurately isolate each person’s voice.
Handling Different Accents and Tones: Podcasts have guests with varied accents and speaking styles. Our system needed to be flexible enough to work with this diversity of voices. No one’s voice needed to get left behind or distorted during the separation process.
Removing Background Noise: On top of overlapping voices, our solution also had to deal with disruptive background noises like street traffic, office chatter, etc. The system had to identify and filter out these unwanted noise intrusions while keeping the speakers’ words clear and pristine.
Speech Separation
Speech separation is the process of isolating individual speakers from a mixed audio signal, a common challenge in audio processing.
The SepFormer model is an optimum choice to perform speech separation using a transformer architecture. SepFormer is a Transformer-based neural network specifically designed for speech separation.
Model Architecture
The Transformer architecture consists of an encoder and a decoder, both are composed of multiple identical layers. Each layer in the encoder and decoder consists of a multi-head self-attention mechanism, followed by a position-wise feed-forward network.
The diagram illustrates the process within the transformer architecture. At the heart of this process, an input signal, denoted as 𝑥, is first passed through an Encoder, which transforms the signal into a higher-level representation, ℎ. This encoded representation is then fed into a Masking Net, where it is multiplied by two different masks, 𝑚1, and 𝑚2, through element-wise multiplication. These masks isolate specific features from the encoded signal that correspond to different sound sources in the mixture.
The outputs from the masking process, now carrying separated audio features, are channeled to a Decoder. The Decoder’s role is to reconstruct the isolated audio signals from these masked features. As a result, the Decoder outputs two separated audio streams, Ŝ1, and Ŝ2, which represent the individual sources originally mixed in the input signal 𝑥. This sophisticated setup effectively separates overlapping sounds, making it particularly useful in environments with multiple speakers.
Our Approach
SpeechBrain Toolkit
SpeechBrain offers a highly flexible and user-friendly framework that simplifies the implementation of advanced speech technologies. Its comprehensive suite of pre-built modules for tasks like speech recognition, speech enhancement, and source separation allows rapid prototyping and model deployment. Additionally, SpeechBrain is built on top of PyTorch, providing seamless integration with deep learning workflows and enabling efficient model training and optimization.
Data Collection and Preparation
LibriMix is an open-source dataset for speech separation and enhancement tasks. Derived from the well-known LibriSpeech corpus, LibriMix consists of two- or three-speaker mixtures combined with ambient noise samples from WHAM!. LibriMix extends its utility by combining various speech tracks to simulate realistic scenarios where multiple speakers overlap, mimicking common real-world environments like crowded spaces or multi-participant meetings.
Using the ‘generate_librimix.sh’ script we generated the LibriMix dataset. The script ‘create_librimix_from_metadata.py’ from the LibriMix repository is designed to create a dataset for speech separation tasks by mixing clean speech sources from the LibriSpeech dataset.
Import Required Libraries: The script starts by importing necessary Python libraries such as os, sys, json, random, and numpy. These libraries provide functionalities for file and system operations, JSON handling, random number generation, and numerical operations.
Define Constants and Parameters: These include paths to the LibriSpeech dataset, the metadata file, and the output directory for the mixed audio files. It also sets parameters for the mixing process, such as the number of sources to mix, the SNR (Signal-to-Noise Ratio) range, and the overlap duration.
Load Metadata: Metadata from the LibriSpeech dataset contains information about the audio files, including their paths, durations, and transcriptions. The metadata is loaded into a Python dictionary for easy access.
Create Mixed Audio Files: The script iterates over the metadata, selecting a subset of audio files to mix. For each iteration, it:
- Selects a random number of sources from the metadata.
- Randomly assigns each source to one of the speakers in the mixed audio.
- Randomly selects an SNR for the mixing process.
- Mixes the selected audio sources, applying the selected SNR and overlap duration.
- Saves the mixed audio file to the output directory.
Generate Metadata for Mixed Audio: This includes the paths to the mixed audio file, the paths to the original audio sources, the SNR used for mixing, and the overlap duration. This metadata is saved in a JSON file, recording how each mixed audio file was created.
Main Function: Orchestrates the above steps. It checks if the output directory exists and creates it if necessary. It loads the metadata, creates the mixed audio files, and generates the metadata for the mixed audio.
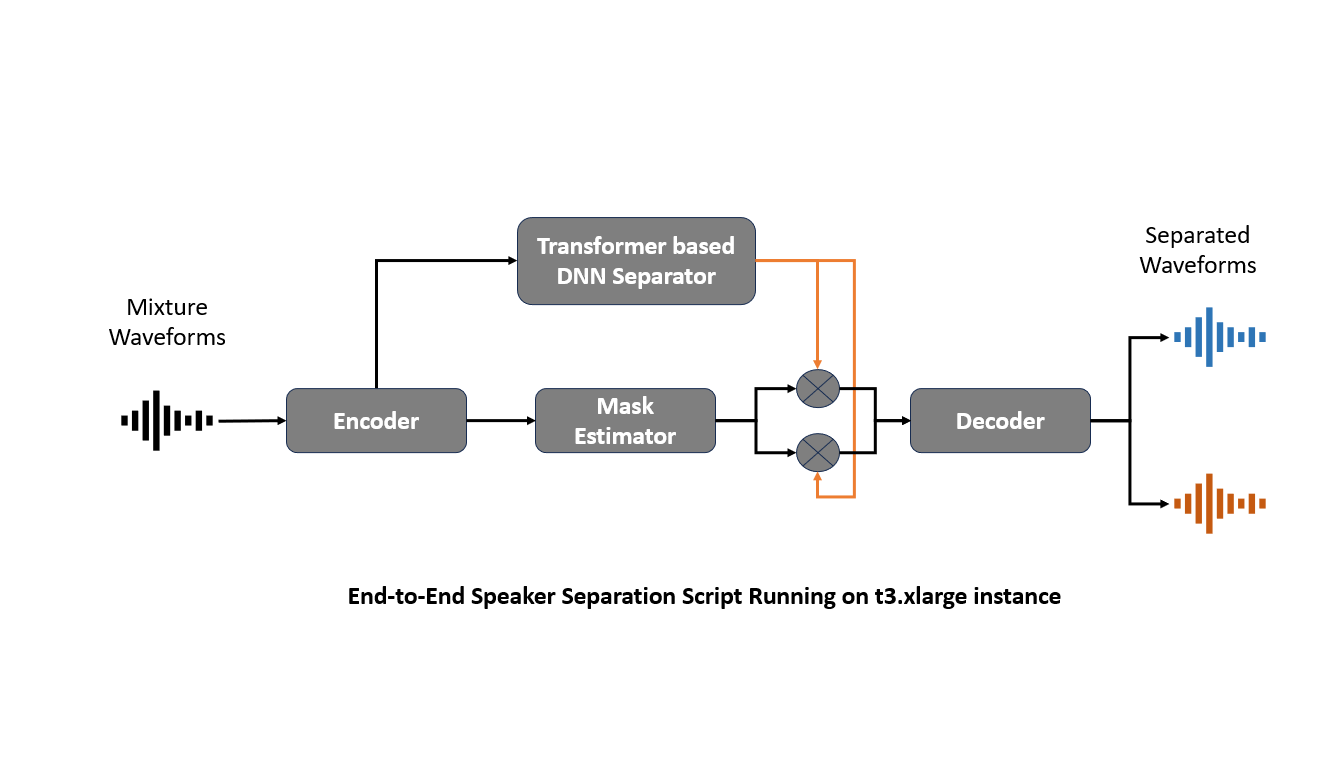
Model Definition
The model architecture for speech separation is built using the PyTorch deep learning library. This step involved setting up the transformer model architecture including layers specifically suited for speech separation tasks.
Encoder
Encoder specifications were as follows:
Kernel Size: 16
Output Channels: 256
Kernel Size: 16 – The convolutional kernel size used in the encoder.
Output Channels: 256 – The number of output channels from the encoder. Corresponds to the dimensionality of the feature maps produced by the encoder.
SBtfintra and SBtfinter
SBtfintra
This component represents the self-attention blocks within the SepFormer model that operate on the intra-source dimension. Specifications:
Num Layers: 8
D Model: 256
Nhead: 8
D Ffn: 1024
Norm Before: True
Num Layers: 8 – The number of self-attention layers in the block.
D Model: 256 – The dimension of the input and output of the self-attention layers.
Nhead: 8 – The number of attention heads, allows the model to focus on different parts of the input simultaneously.
D Ffn: 1024 – The dimension of the feed-forward network within each self-attention layer.
Norm Before: True – Layer normalization is applied before the self-attention layers, which helps stabilize the training process.
SBtfinter
This component represents the self-attention blocks operating on the inter-source dimension similar to SBtfintra. It is configured with the same parameters as SBtfintra, indicating that intra-source and inter-source dimensions are processed with the same structure.
MaskNet
MaskNet specifications:
Num Spks: 3
In Channels: 256
Out Channels: 256
Num Layers: 2
K: 250
Num Spks: 3 – The number of sources to separate, which corresponds to the number of masks the model will learn to produce.
In Channels: 256 – The number of input channels to the mask network, matching the output channels of the encoder).
Out Channels: 256 – The number of output channels from the mask network corresponds to the dimensionality of the masks produced by the model.
Num Layers: 2 – The number of layers in the mask network, which processes the encoder’s output to produce the masks.
K: 250 – The size of the masks produced by the model determines the resolution of the separated sources.
Decoder
Decoder specifications:
In Channels: 256
Out Channels: 1
Kernel Size: 16
Stride: 8
Bias: False
In Channels: 256 – The number of input channels to the decoder, matches the output channels of the mask network.
Out Channels: 1 – The number of output channels from the decoder corresponds to the dimensionality of the separated audio sources.
Kernel Size: 16 – The convolutional kernel size used in the decoder is similar to the encoder.
Stride: 8 – The convolutional layers stride in the decoder affects the spatial resolution of the output.
Bias: False – Indicates that no bias is applied to the convolutional layers in the decoder.
Training Process
Prepare Data Loaders: Training, validation, and test datasets are wrapped in DataLoader instances that handle batching, shuffling, and multiprocessing for loading data. The ‘LibriMixDataset’ class loads the LibriMix dataset, a speech signals mixture. The dataset is divided into training and validation sets. The ‘DataLoader’ class is then used to load the data in batches.
Training Parameters:
- Number of Epochs: 200
- Batch Size: 1
- Learning Rate (lr): 0.00015 – The learning rate for the Adam optimizer
- Gradient Clipping Norm: 5
- Loss Upper Limit: 999999
- Training Signal Length: 32000000
- Dynamic Mixing: False
- Data Augmentation: Speed perturbation, frequency drop, and time drop settings
Evaluation
After training, the model is evaluated to determine its performance by running it against a test dataset and logging the output. We then defined the Scale-Invariant Signal-To-Noise Ratio (SI-SNR) loss with a PIT wrapper, suitable for the source separation task.
Inference
Used the trained model to predict new data to separate speech from overlapping conversations and background noise.
Measurable Impact
Time Savings: Introducing our advanced speech separation system has improved the efficiency of podcast production and reduced the editing time by 17%.
Cost Reduction: This enhanced efficiency and reduced editing time lowered the operational costs by 15%.
Enhanced Listener Engagement: There has been an 8% increase in listener engagement.
Reduced Communication Errors: The deployment of our system led to a 14% reduction in communication errors.
Improved Audio Quality: Overall audio and voice quality improved by 12%, enhancing the listening experience.
Reduced False Positives: The system has achieved a 5% decrease in false positives in voice detection, ensuring a more accurate and enjoyable listening experience.
Conclusion
By leveraging advanced speech processing toolkits and developing a transformer-based deep-learning model, our team at Rudder Analytics created a speech separation system that significantly improved audio quality and listener engagement. As speech processing, speech engineering, and Natural Language Processing technologies continue to evolve, we can expect even more innovative solutions that will redefine the way we create, consume, and engage with podcasts and other audio content. As audio content consumption grows across platforms, the demand for clear, intelligible audio will only increase. We invite businesses, creators, and audio professionals to explore how our speech separation technology can elevate their audio output and deliver unparalleled listening experiences to their audiences.
Elevate your projects with our expertise in cutting-edge technology and innovation. Whether it’s advancing speech processing capabilities or pioneering new tech frontiers, our team is ready to collaborate and drive success. Join us in shaping the future—explore our services, and let’s create something remarkable together. Connect with us today and take the first step towards transforming your ideas into reality.
Drop by and say hello! Website LinkedIn Facebook Instagram X GitHub
Voice-Controlled Amenities for Enhanced Hotel Guest Experience
Voice-Controlled Amenities for Enhanced Hotel Guest Experience
In the hospitality sector, delivering exceptional guest experiences is a top priority. One hotel chain recognized an opportunity to enhance its offerings through voice-enabled technology. They partnered with us to implement a wake word detection system and voice-activated concierge services. The goal was to elevate convenience and satisfaction by enabling guests to control room amenities like lighting, temperature, and entertainment via voice commands. This technical blog post will dive into the details of the wake word detection system developed by Rudder Analytics, exploring the approaches used to ensure accurate speech recognition across diverse acoustic environments, user voices, and speech patterns.
Wake Word Detection
Wake word detection, also known as keyword spotting, is a critical component of voice-enabled systems that allow users to activate and interact with devices or applications using predefined voice commands. This technology is crucial in various applications, including virtual assistants, smart home devices, and voice-controlled interfaces.
The primary objective of wake word detection is to continuously monitor audio streams for the presence of a specific wake word or phrase. After detecting the wake word, the system activates and listens for subsequent voice commands or queries. Effective wake word detection systems must balance accuracy, computational efficiency, and power consumption.
A brief overview of the process:
The process begins when the user speaks to the device/application. The words are captured as an audio input.
Next comes the feature extraction where specific characteristics are extracted from the sound of the user’s voice that will help recognize the wake word.
Now, the device/application turns these features into embedding representation, like a unique digital fingerprint that represents the wake word’s sound pattern.
This is where the pre-trained model comes into play. Before the device/application listens to the user, it is trained on many examples to learn what the wake word sounds like.
This model is fine-tuned with target keyword examples (the actual wake words it needs to listen for) and non-target examples (all the other words that aren’t the wake word).
The fine-tuned model then captures only the wake word while neglecting the other non-target words.
Challenges to Tackle
The main challenge was to develop a wake word detection system that accurately recognizes specific commands within a continuous audio stream. This task was complicated by the need for the system to perform reliably across various acoustic settings, from quiet rooms to those with background noise or echoes. Additionally, the system had to be versatile enough to recognize spoken commands by a wide array of users, each with their unique voice, accent, and speech pattern.
Crafting Our Solution
Few-Shot Transfer Learning Approach
Few-shot transfer learning is a technique that can enable machine learning models to quickly adapt to new tasks using only a limited number of examples. The approach builds upon extensive prior training on related but broad tasks, allowing models to leverage learned features and apply them to new, specific challenges with minimal additional input.
This strategy is particularly valuable in scenarios where data is scarce. By enhancing model adaptability and efficiency, this technique offers a realistic solution to data scarcity in natural language processing. The ability of few-shot transfer learning to empower machine learning models to generalize from limited data has significant practical applications, making it an increasingly popular research topic in the field of artificial intelligence.
Model Fine Tuning
1. Starting With a Foundation
Our system begins with a pre-trained multilingual embedding model. This is a base model that’s already been trained on a vast array of languages and sounds, giving it a broad understanding of speech patterns.
Pre-trained Multilingual Embedding Model
Our approach leveraged a Deep Neural Network (DNN)-based, pre-trained multilingual embedding model. This model was initially trained on 760 frequent words from nine languages, drawing from extensive datasets such as:
MLCommons Multilingual Spoken Words: Contains more than 340,000 keywords, totaling 23.4 million 1-second spoken examples (over 6,000 hours)
Common Voice Corpus: 9,283 recorded hours with demographic metadata like age, sex, and accent. The dataset consists of 7,335 validated hours in 60 languages.
Google Speech Commands for background noise samples: 8.17 GiB audio dataset of spoken words designed to help train and evaluate keyword spotting systems.
This rich training background laid a foundation for the system’s language and accent inclusivity.
2. Selective Hearing
The fine-tuning process involves teaching the model to focus on a small set of important sounds – the wake words. Fine-tuning the model for a new wake word using few-shot transfer learning is achieved by updating the model’s weights using a small dataset of audio recordings containing the new wake word.
With the hotel’s custom needs in mind, we fine-tuned the model with just five custom target keyword samples as training data. This method allowed us to fine-tune a five-shot keyword spotting context model, enabling the pre-trained model to generalize over new data categories swiftly.
3. Distinguishing the Target
It’s not just about knowing the wake word but also about knowing what it’s not. An unknown keywords dataset of 5,000 non-target keyword examples was used to maintain the ability of the few-shot model to distinguish between the target keyword and non-target keywords.
4. Tailored Adjustments
The pre-trained model was adjusted incrementally, learning to recognize the wake word more accurately from the examples provided. This involved tweaking the internal settings, or parameters, of the model to minimize errors. The fine-tuning process typically employs a variant of stochastic gradient descent (SGD) or other optimization algorithms.
5. Testing and Retesting
After each adjustment, the model was tested to see how well it can distinguish the wake word from other sounds. It’s a cycle of testing, learning, and improving.
6. Optimizing for Real World Use
During fine-tuning, the model was introduced to variations of the wake word as it might be spoken in different accents, pitches, or speech speeds, ensuring the model can recognize the wake word in diverse conditions. This was done by using techniques like data augmentation and noise addition.
7. Reducing False Triggers
A crucial part of fine-tuning is to reduce false positives—times when the device wakes up but shouldn’t. This involves adjusting the model so that it becomes more discerning and able to tell apart similar words or sounds from the actual wake word.
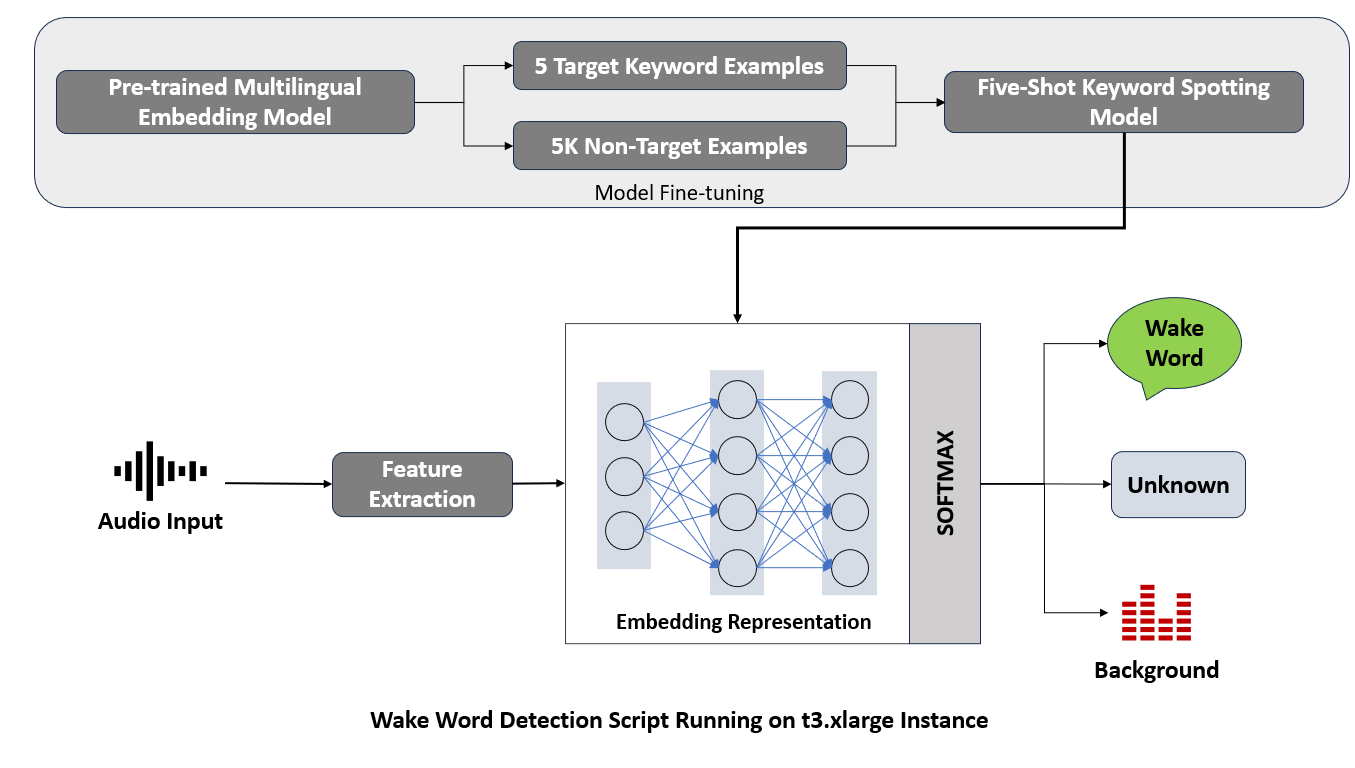
Fine-tuned Wake Word Detection Model
1. Audio Input and Feature Extraction
At the start of the wake word detection pipeline, audio input is received and passed through a feature extraction process. This step is crucial for transforming raw audio waveforms into a structured format that the neural network can interpret. Feature extraction algorithms focus on isolating the most relevant aspects of the audio signal, such as frequency and amplitude, which are informative of the content within the audio.
2. Neural Network and Embedding Representation
The extracted features are then input into a neural network, which acts as the engine of the wake word detection system. The network maps the features to an embedding space, where the learned representations are optimized to cluster target wake words close together while distancing them from non-target sounds and words.
3. The Softmax layer
The use of a softmax layer is standard in classification tasks. However, in the context of wake word detection, the softmax layer presents a unique challenge. It needs to classify inputs into one of three categories: the wake word, unknown words, or background noise. The softmax layer must be finely tuned to ensure that it can confidently distinguish between these categories, which is critical for reducing both false positives and negatives.
4. Real-time Processing
An efficient sliding window mechanism was implemented to enable the real-time analysis of continuous audio streams, ensuring prompt system responsiveness with minimal latency.
5. Deployment on a Cloud Instance
Once the model is trained and validated, it’s deployed to a cloud-based service running on a t3.xlarge instance. This selection of cloud computing resources ensures that the wake word detection script has access to high performance and scalability to handle real-time audio processing without significant latency.
Measurable Impact and Beyond
The implementation of this system had a clear impact, achieving an accuracy of 97% in wake word detection and a remarkable 99.9% uptime during stress testing and performance evaluations. This reliability ensured the system’s scalability and dependability, critical factors in a hotel environment where downtime can significantly affect guest satisfaction.
The most telling outcome was the 23% increase in guest satisfaction scores following the system’s implementation. This surge in guest approval underscored the system’s effectiveness in enhancing the overall stay experience, affirming the value of integrating AI and ML technologies in service-oriented industries.
Our wake word detection system ensured high accuracy, decreased the occurrence of false positives, and operated with reduced latency, facilitating immediate and correct command detection, and thereby considerably improving the overall user experience.
Conclusion
In wrapping up this project on voice-controlled hotel room amenities, it’s clear that our technical efforts and the application of AI and ML have significantly improved how customer service can be delivered. This work highlights the practical benefits of leveraging advanced technologies to make everyday interactions more user-friendly and efficient. At Rudder Analytics, our focus remains on exploring the potential of AI and ML to contribute to progress and achieve high standards in various fields.
Elevate your projects with our expertise in cutting-edge technology and innovation. Whether it’s advancing voice-controlled system capabilities or pioneering new tech frontiers, our team is ready to collaborate and drive success. Join us in shaping the future—explore our services, and let’s create something remarkable together. Connect with us today and take the first step towards transforming your ideas into reality.
Drop by and say hello! Website LinkedIn Facebook Instagram X GitHub